使用 reComputer Jetson 进行工地安全帽检测
介绍
在工业或建筑工地等工作环境中,安全帽是必需的,能够保护人们的头部免受掉落物体、与其他物体碰撞、碎片、雨水和电击造成的伤害。 它提高了安全性,但有时人们低估了其个人和工业上的重要性。 因此,基于视频的监控系统来检测安全帽可以成为解决这一安全问题的优化方案。
因此,感谢Louis Moreau和Mihajlo Raljic,我们提供这个基础项目,我们将训练一个嵌入式机器学习模型来检测安全帽,并将其部署到 Jetson Nano。 Jetson NX 和 Jetson AGX 都受到支持。
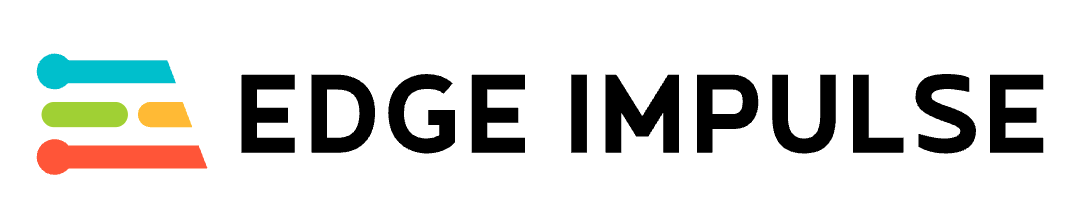
开始
Edge Impulse使开发人员能够创建下一代智能设备解决方案,利用嵌入式机器学习。边缘的机器学习将使今天由于成本、带宽或功耗限制而被丢弃的99%的传感器数据得到有价值的利用。 在这里,我们将应用 Edge Impulse 来训练一个嵌入式机器学习模型。
硬件
所需硬件
在这个项目中,所需的设备如下所示:
- NVIDIA Jetson Nano 或 NVIDIA Xavier NX 或 NVIDIA Xavier AGX
- 个人电脑
- USB 摄像头
- HDMI 显示屏
硬件设置
个人电脑和 NVIDIA Jetson Nano 应该开机并连接到互联网。 建议将 NVIDIA Jetson Nano 设置为个人电脑。
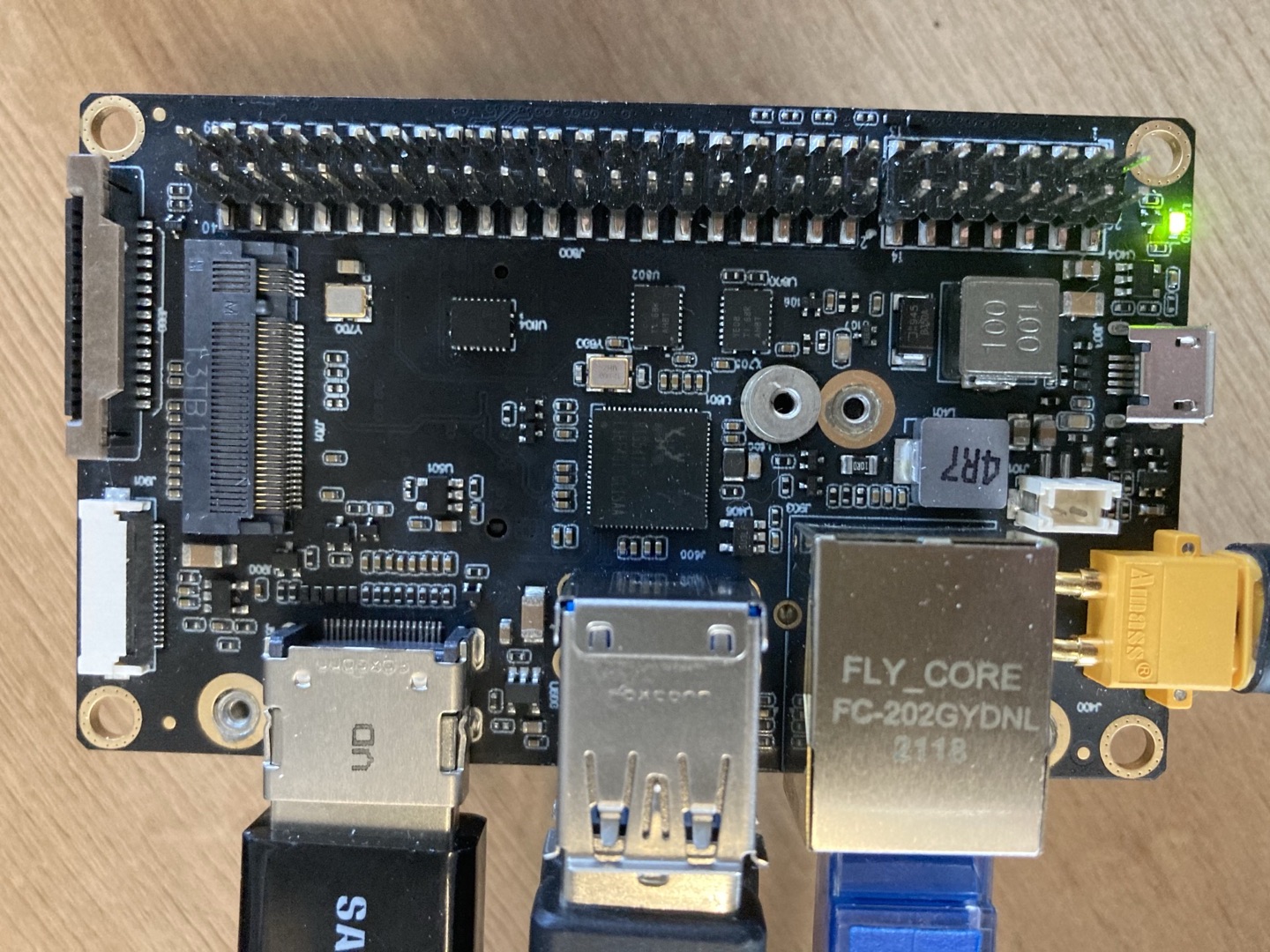
软件
- Edge Impulse
- Ubuntu 系统 用于 NVIDIA Jetson Nano
在这里,我们将训练一个嵌入式机器学习模型来检测安全帽。有几种方法可以为此做出贡献。
准备工作
在我们开始项目之前,有一些准备工作需要先完成。
- 步骤 1. 打开 Edge Impulse 网站,并注册一个账户。
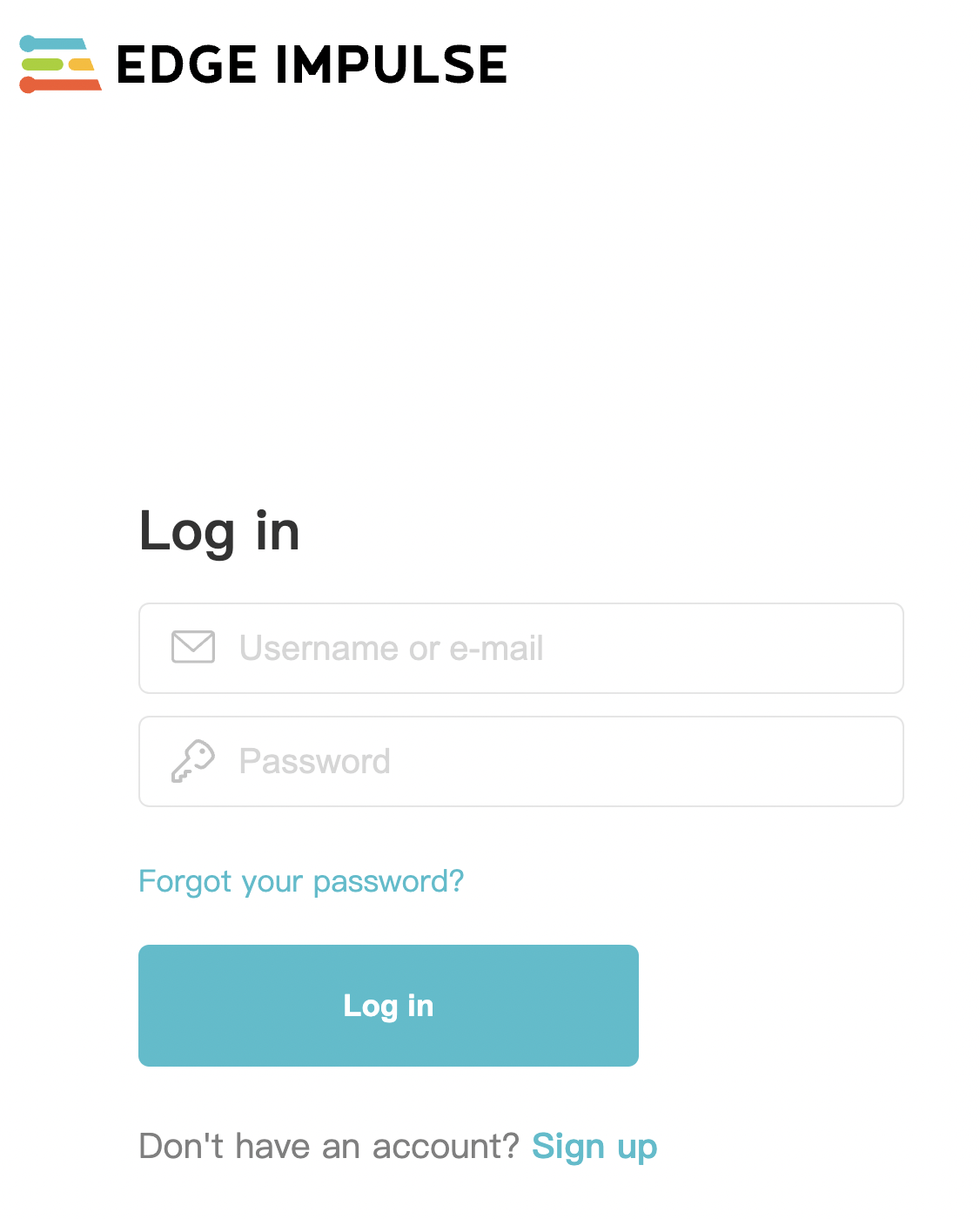
- 步骤 2. 点击 "创建新项目" 并输入项目名称。
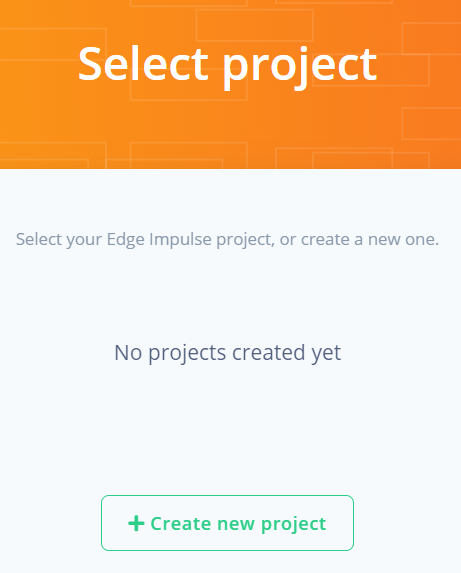
在这里我们输入 "安全帽检测"。
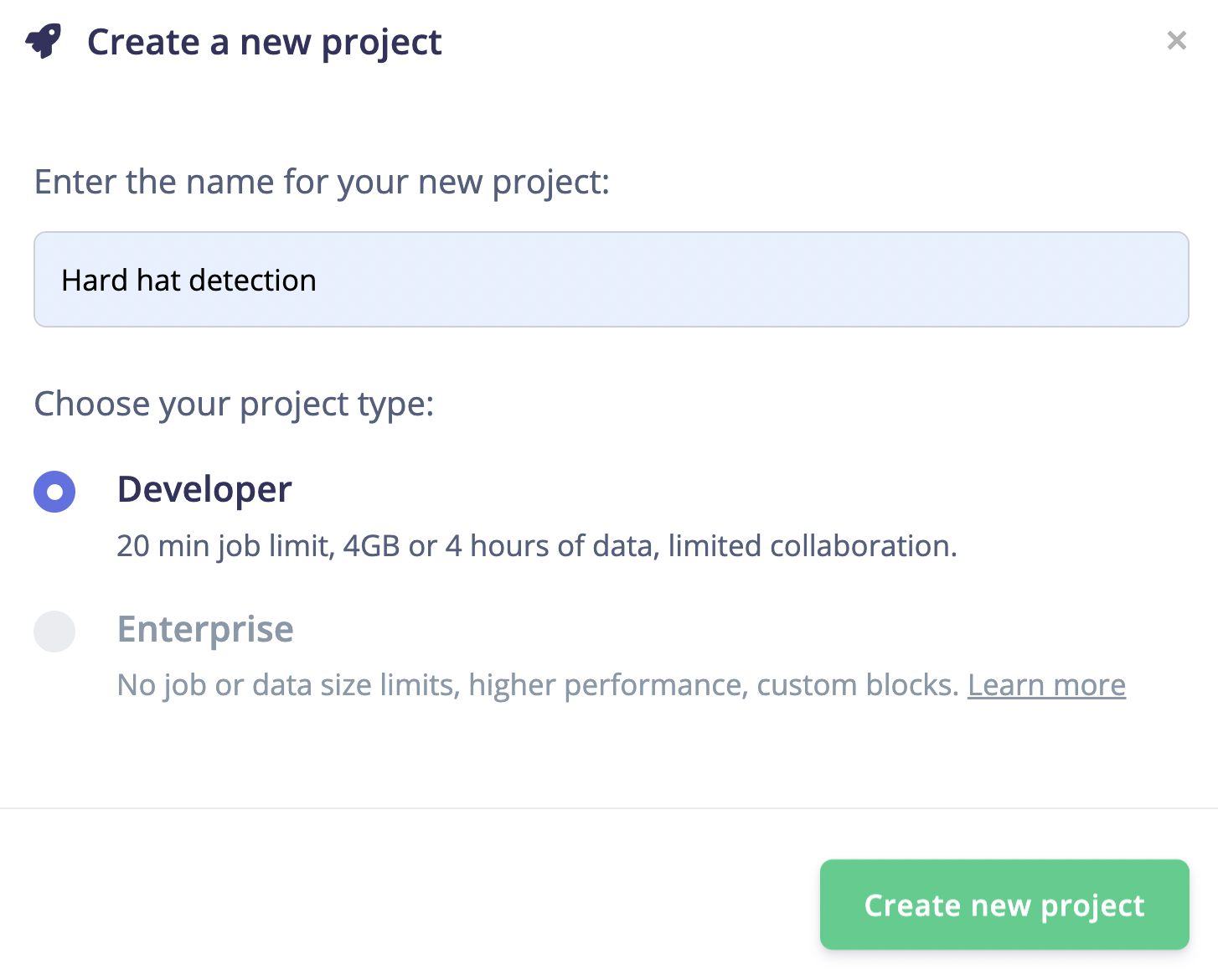
- 步骤 3. 我们将训练一个嵌入式机器学习模型来检测安全帽,因此在这里应该选择 "图像" 选项。
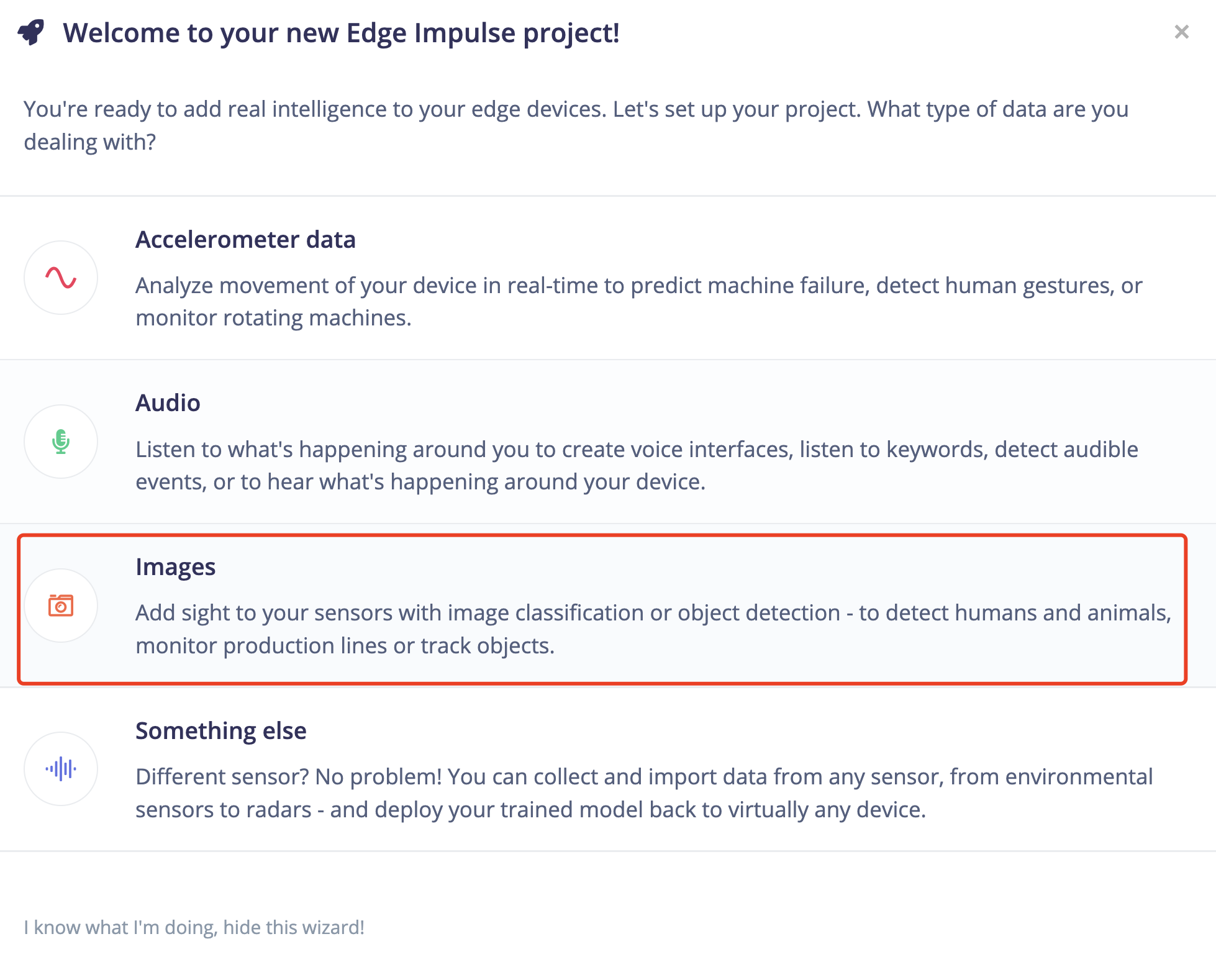
- 步骤 4. 将配置设置为 "分类多个对象(目标检测)"。
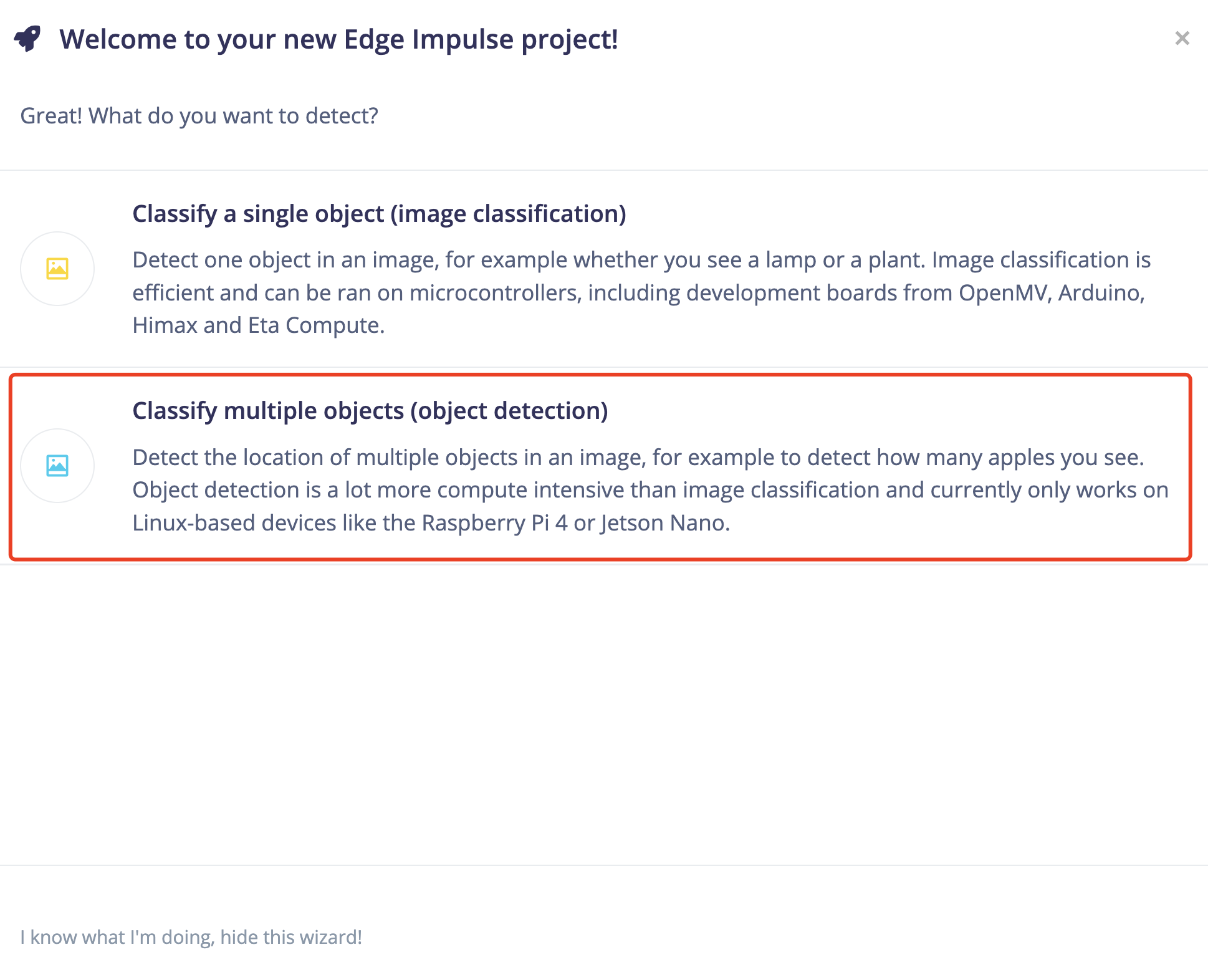
现在我们可以开始这个项目了。
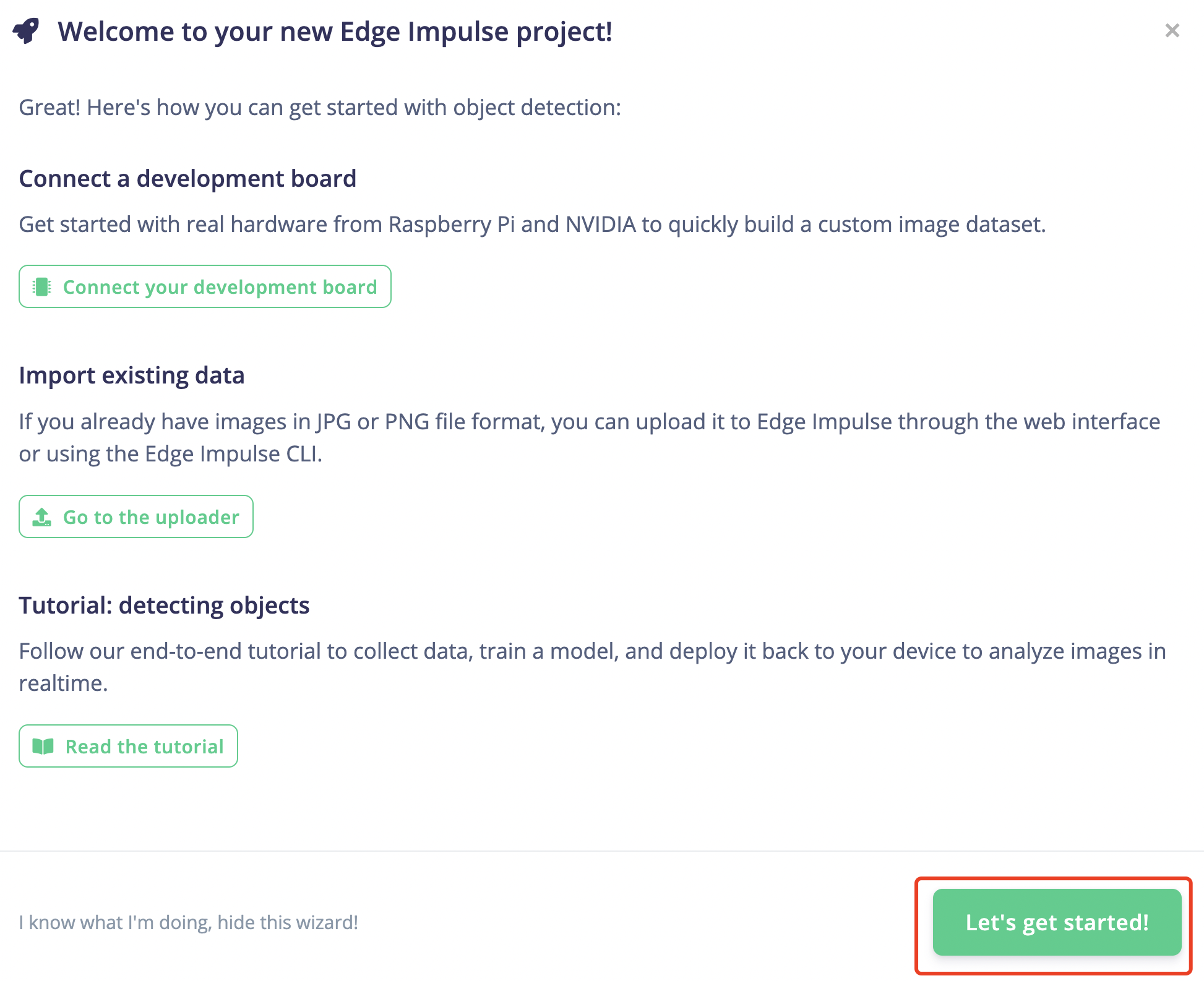
安全帽检测机器学习模型训练
基于输入公共数据集的机器学习模型训练
Edge Impulse 提供了几种收集数据的方法。 首先,我们将把公共数据上传到网站,并尝试开发一个嵌入式机器学习。
- 步骤 1. 在左侧栏中选择"数据采集"页面并收集数据。
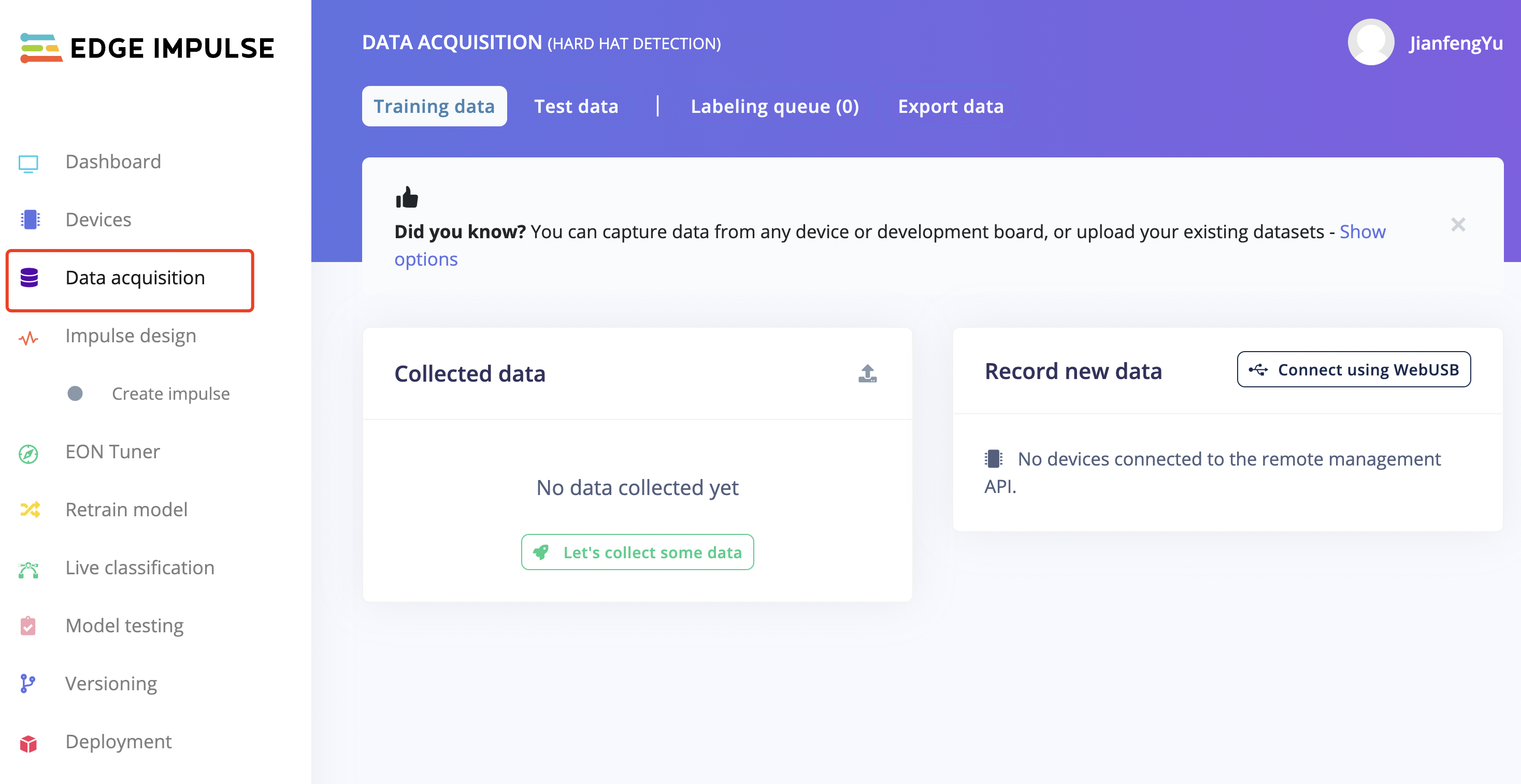
- 步骤 2. 选择并下载数据集来自 Flickr-Faces-HQ 数据集 GitHub.
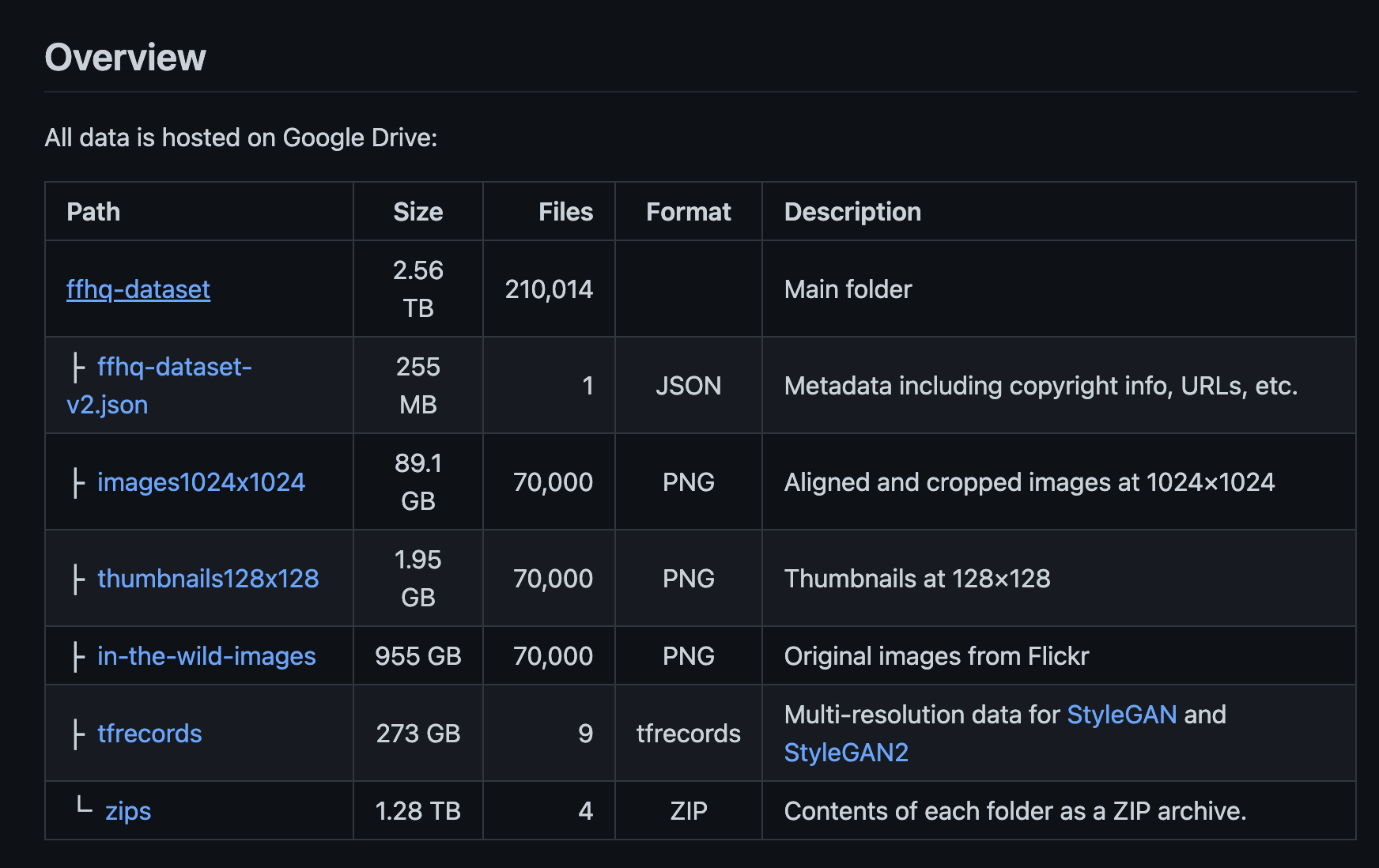
点击"上传数据"按钮,在"数据采集"页面上传下载的数据集。
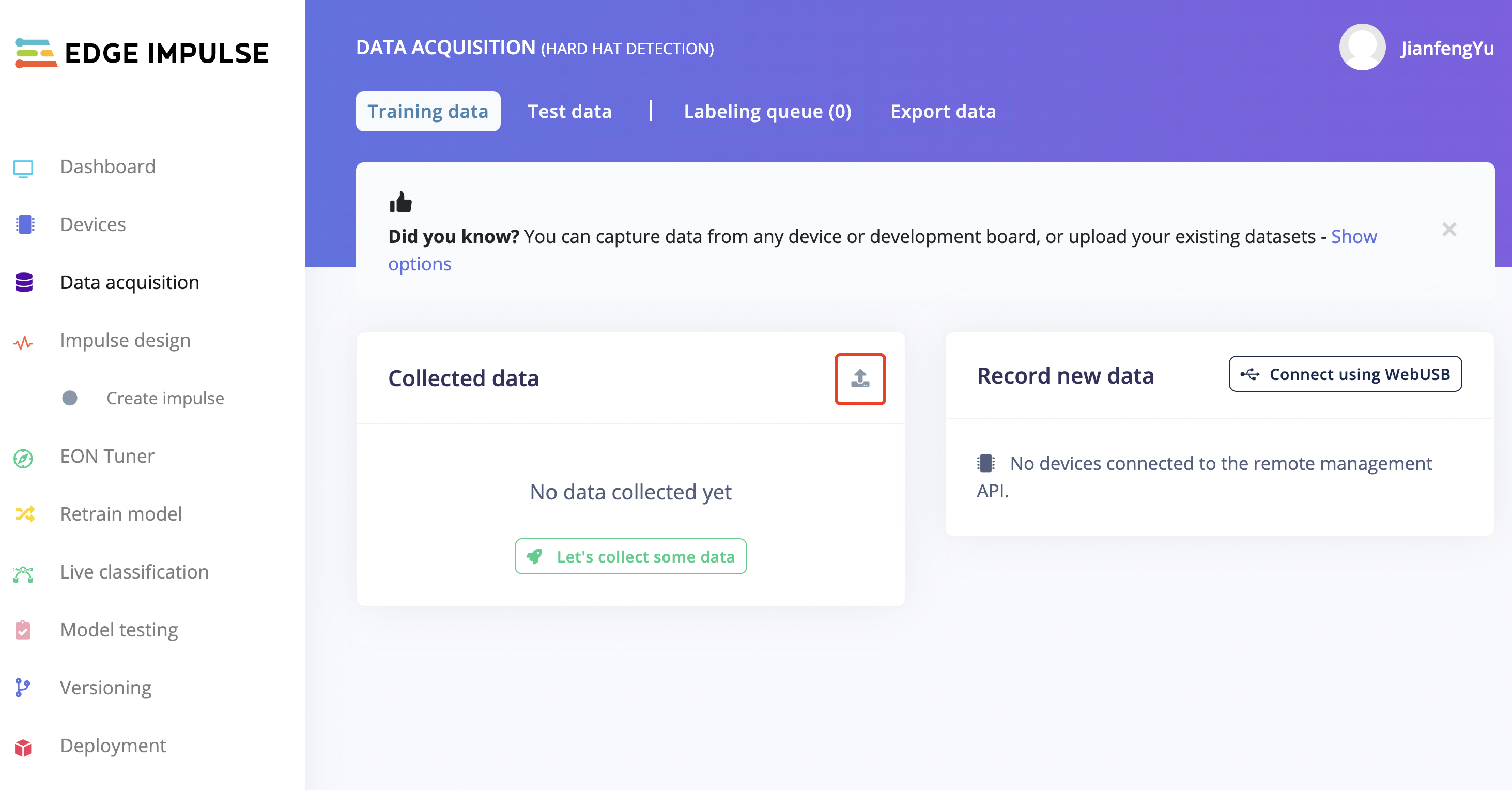
可选择以数据采集格式(CBOR、JSON、CSV)上传现有数据,或以WAV、JPG或PNG文件格式上传。
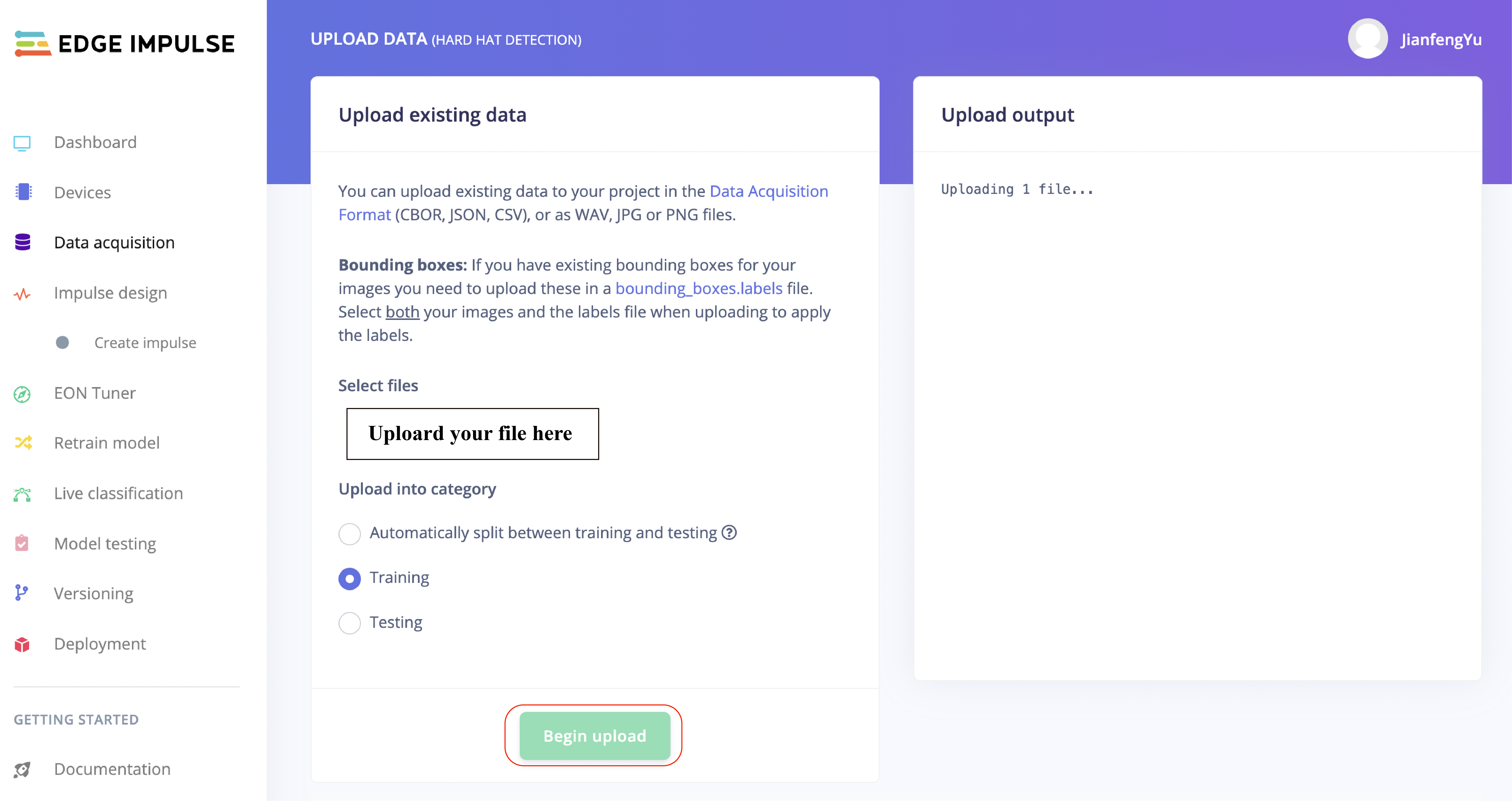
- 步骤 3. 上传后,收集的数据将被填充带标签的图像。 继续点击页面左侧的"冲动设计"。
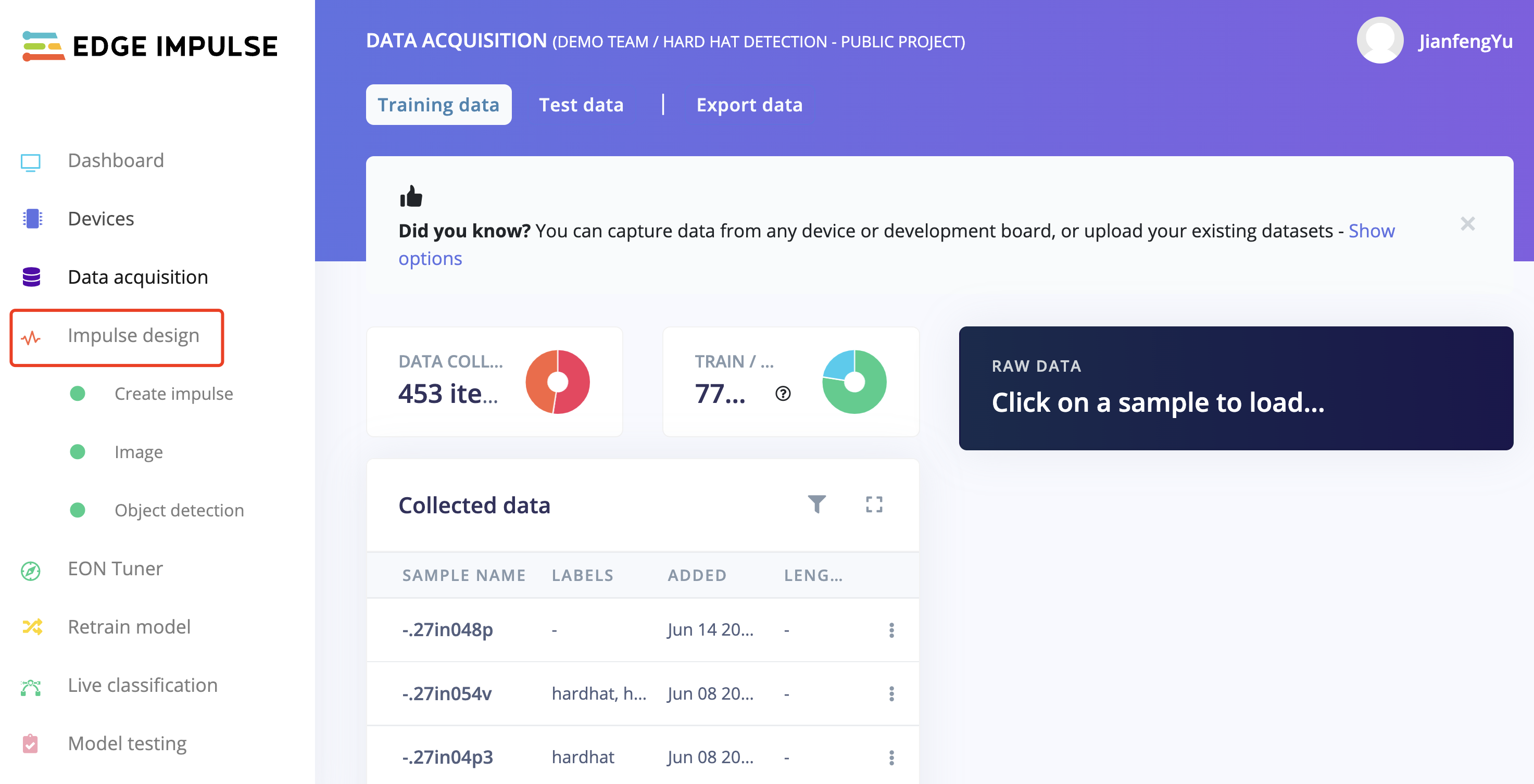
- 步骤 4. 选择合适的图像处理块和图像学习块并保存冲动。
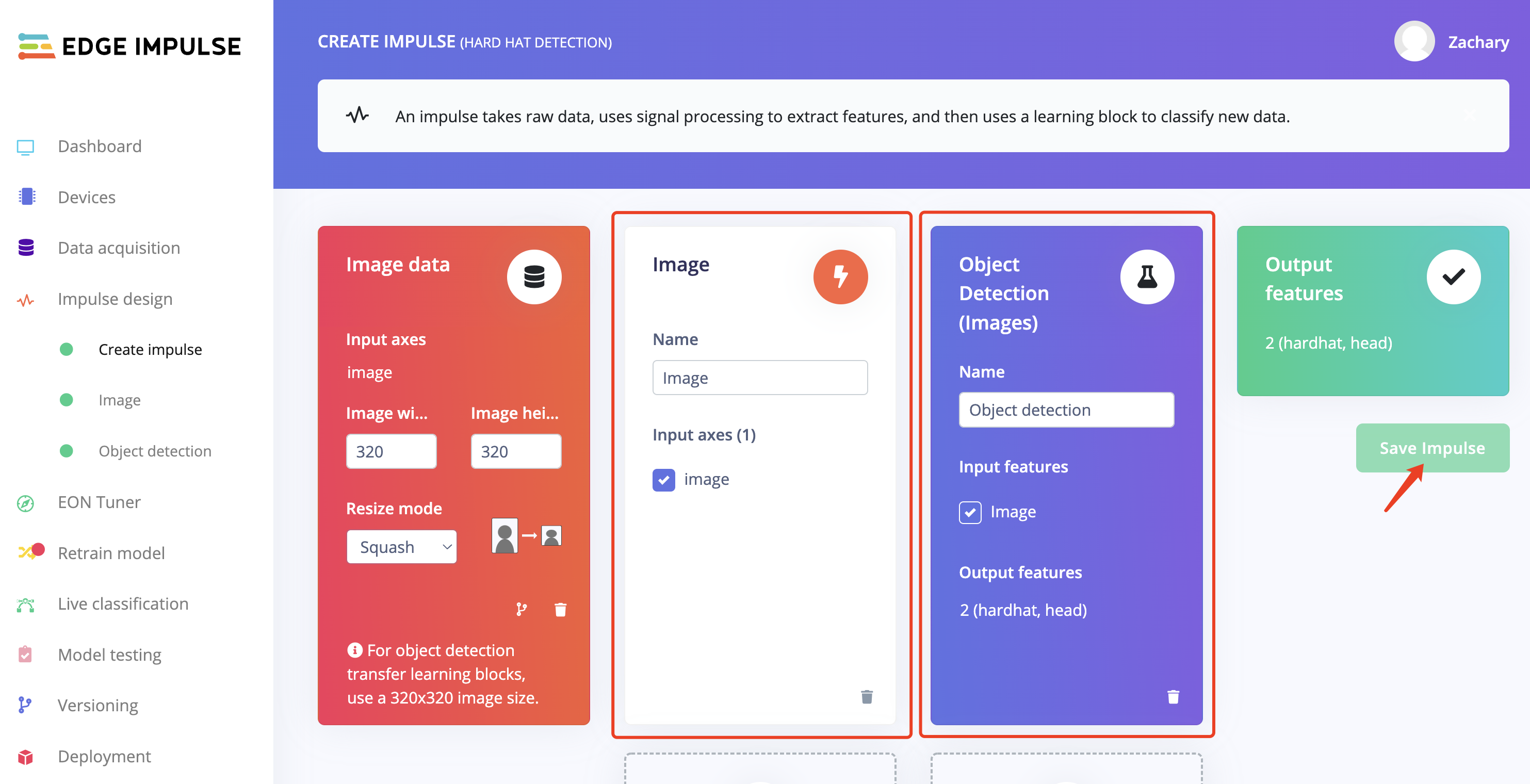
- 步骤 5. 点击页面左侧的"图像"。
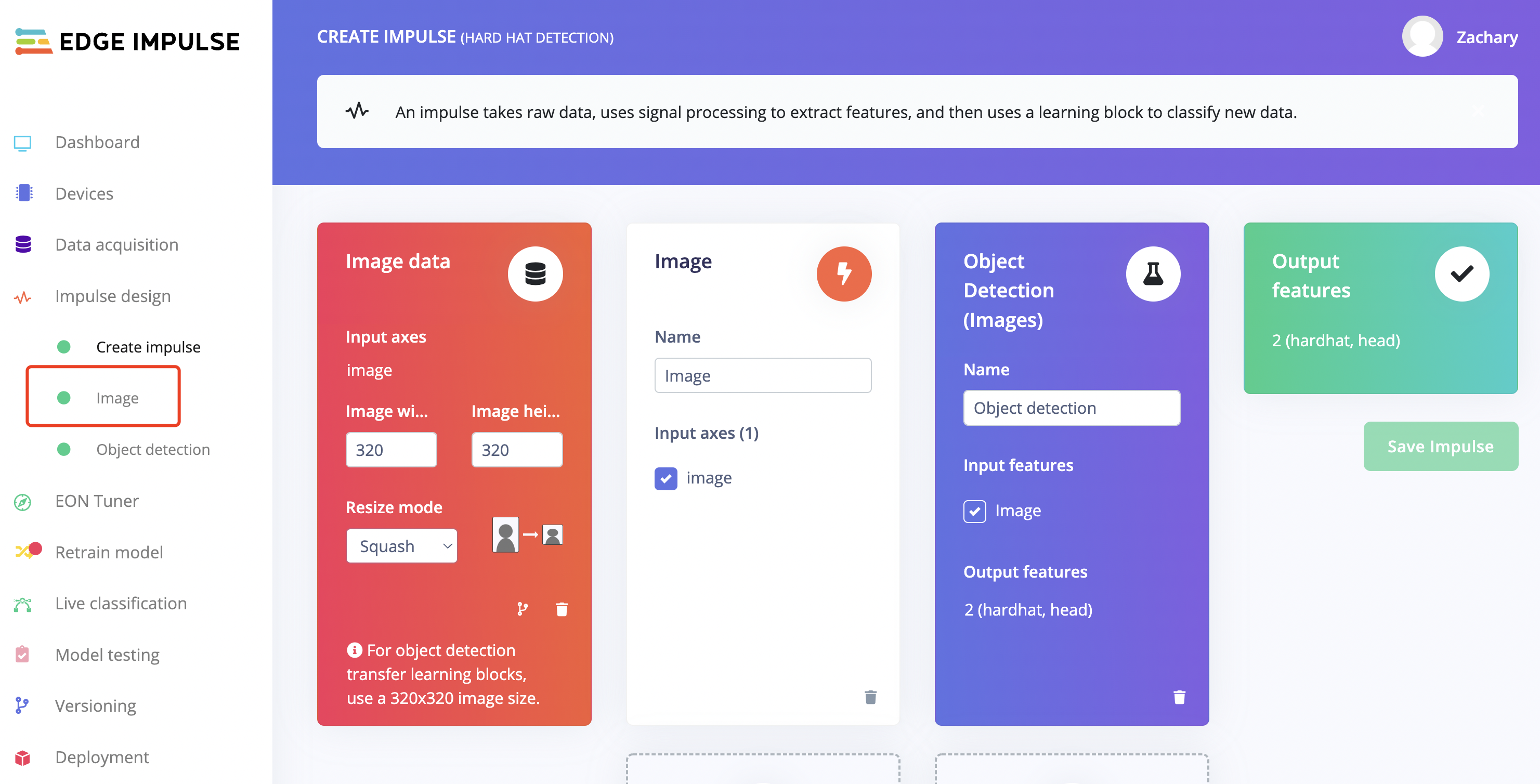
配置为"GRB"并点击"保存参数",页面将自动转到"生成特征"网站。
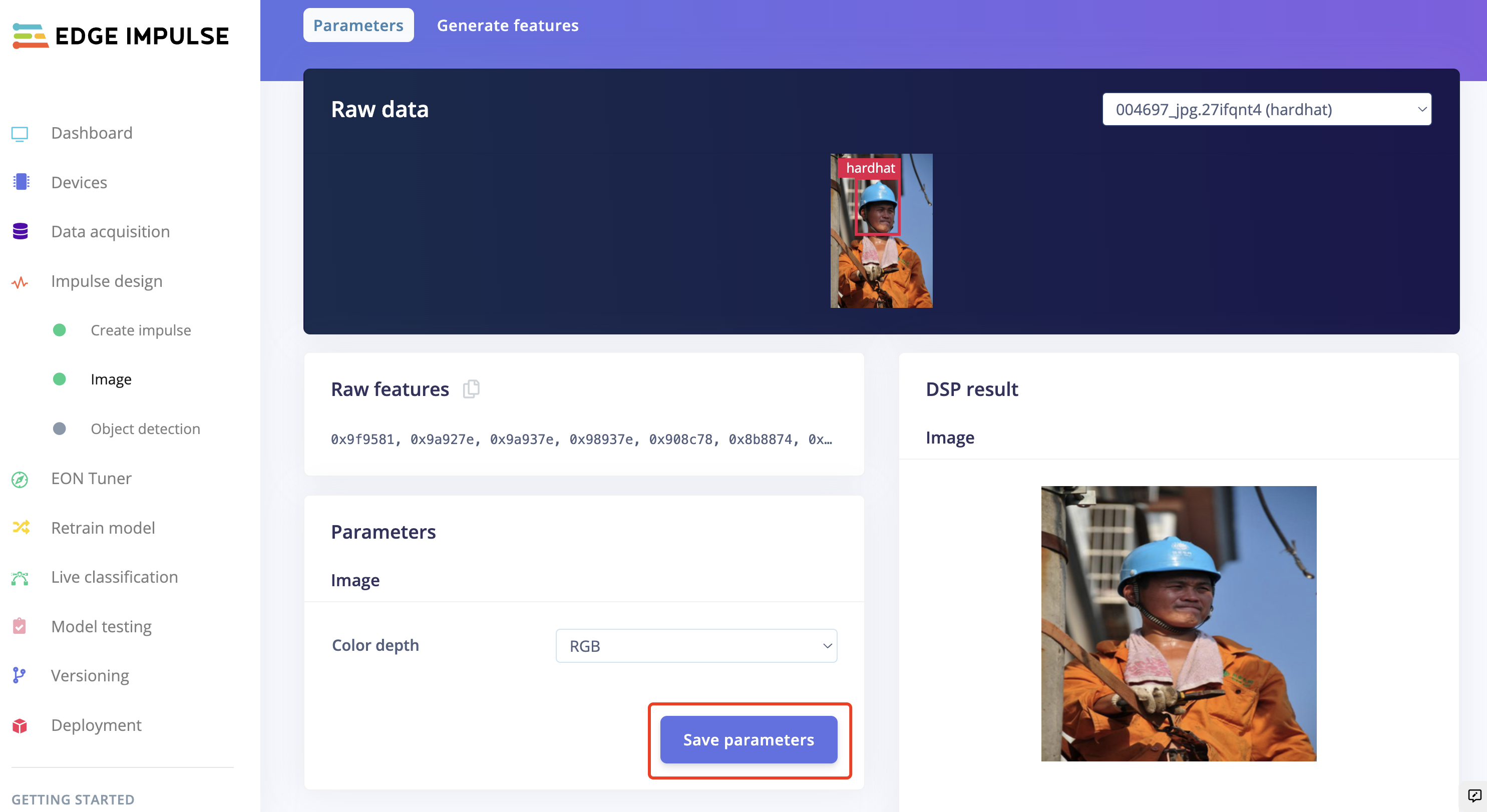
我们能够生成特征。
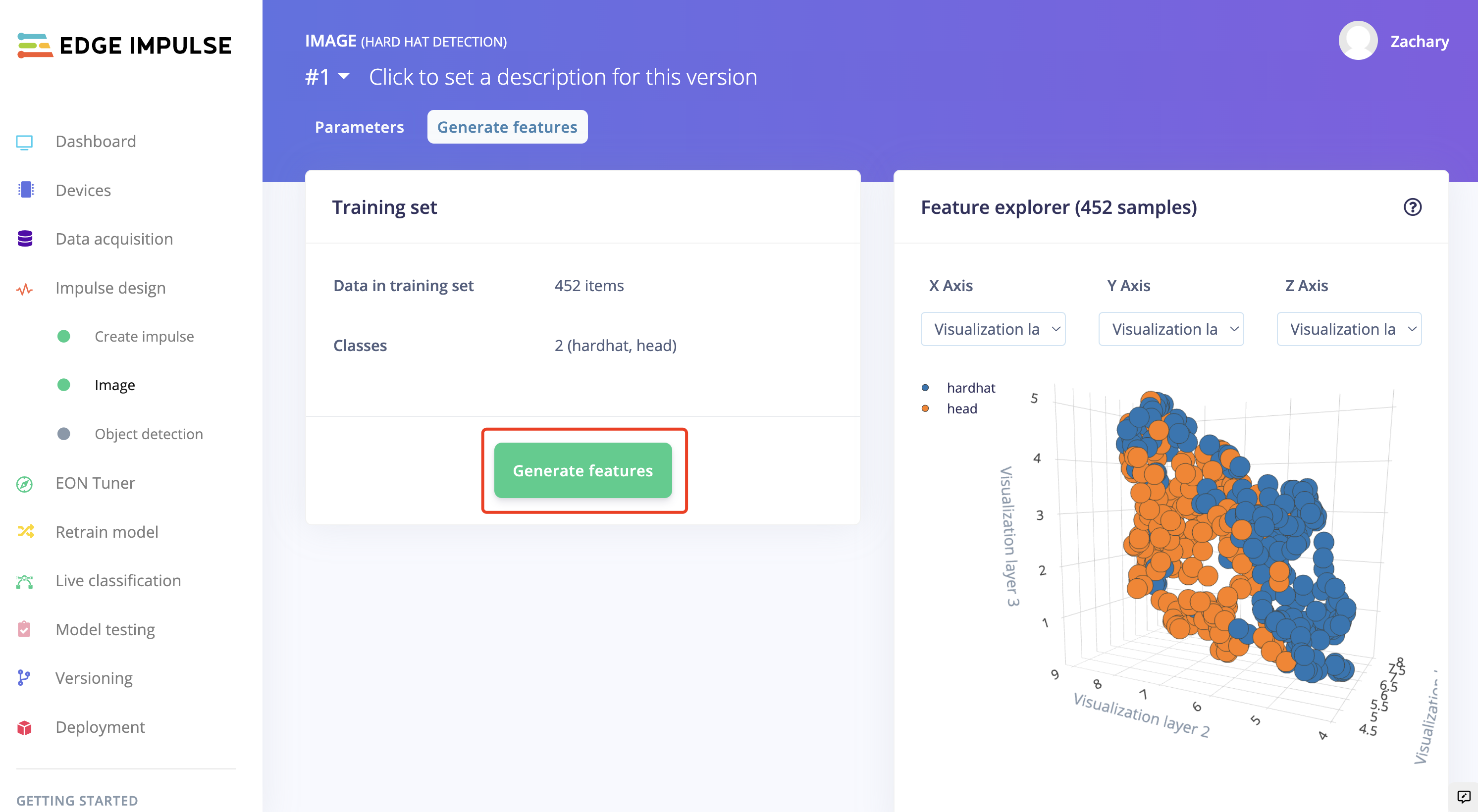
- 步骤 6. 当出现 "任务完成" 时,点击页面左侧的 "目标检测"。
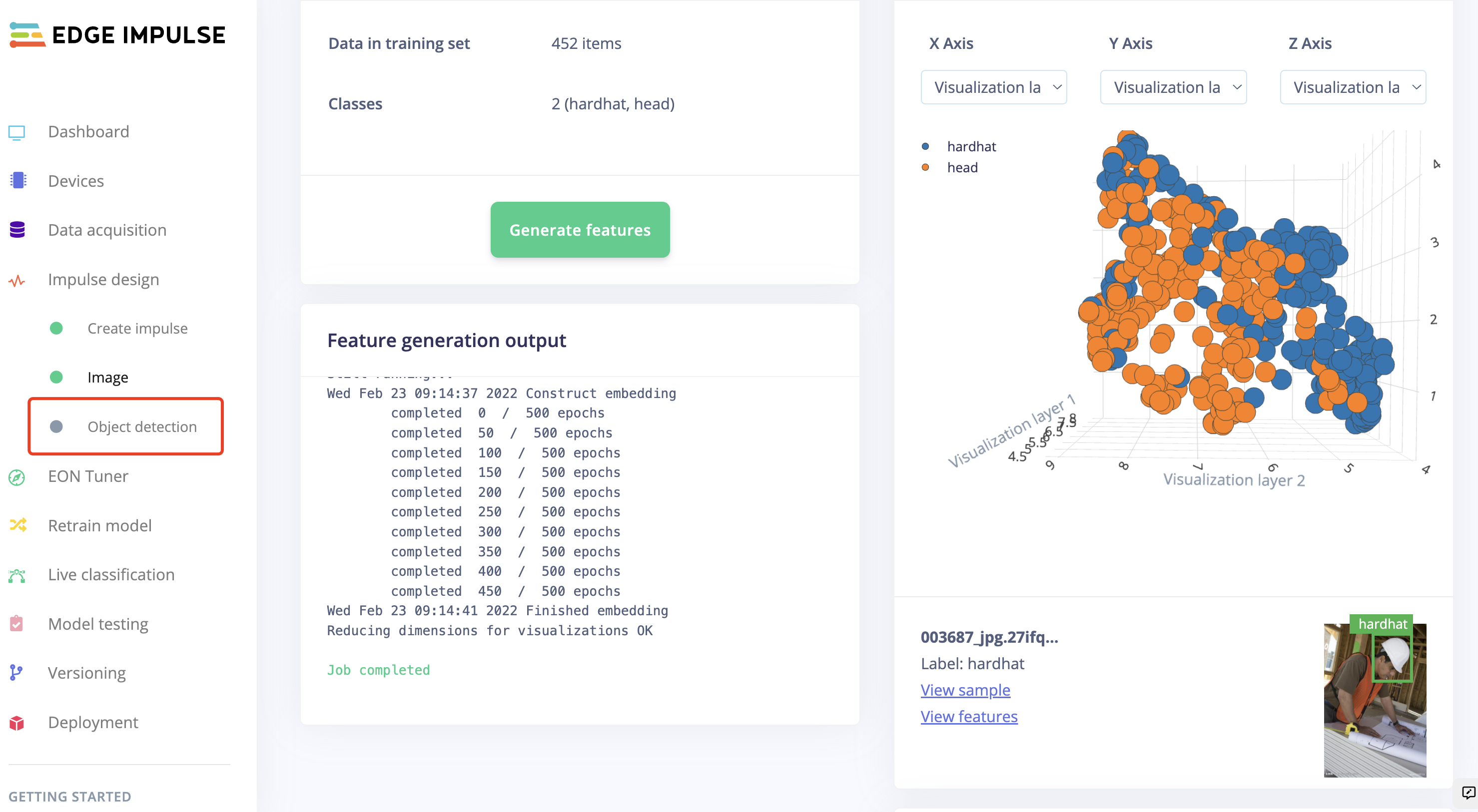
点击 "开始训练",让 Edge Impulse 根据生成的特征训练模型。
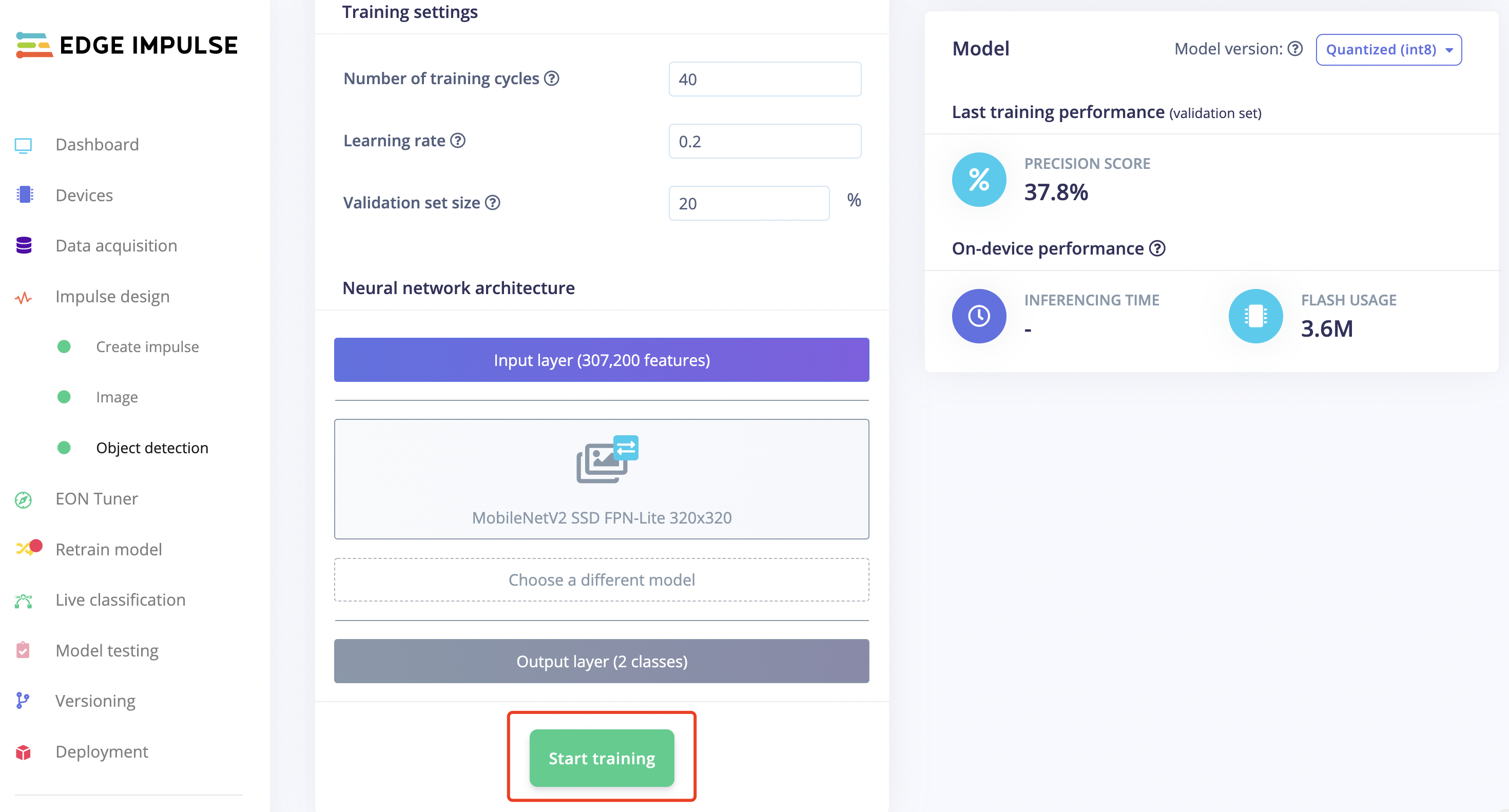
- 步骤 7. 一旦出现 "任务完成",点击 "模型测试" 来检查模型的工作情况。
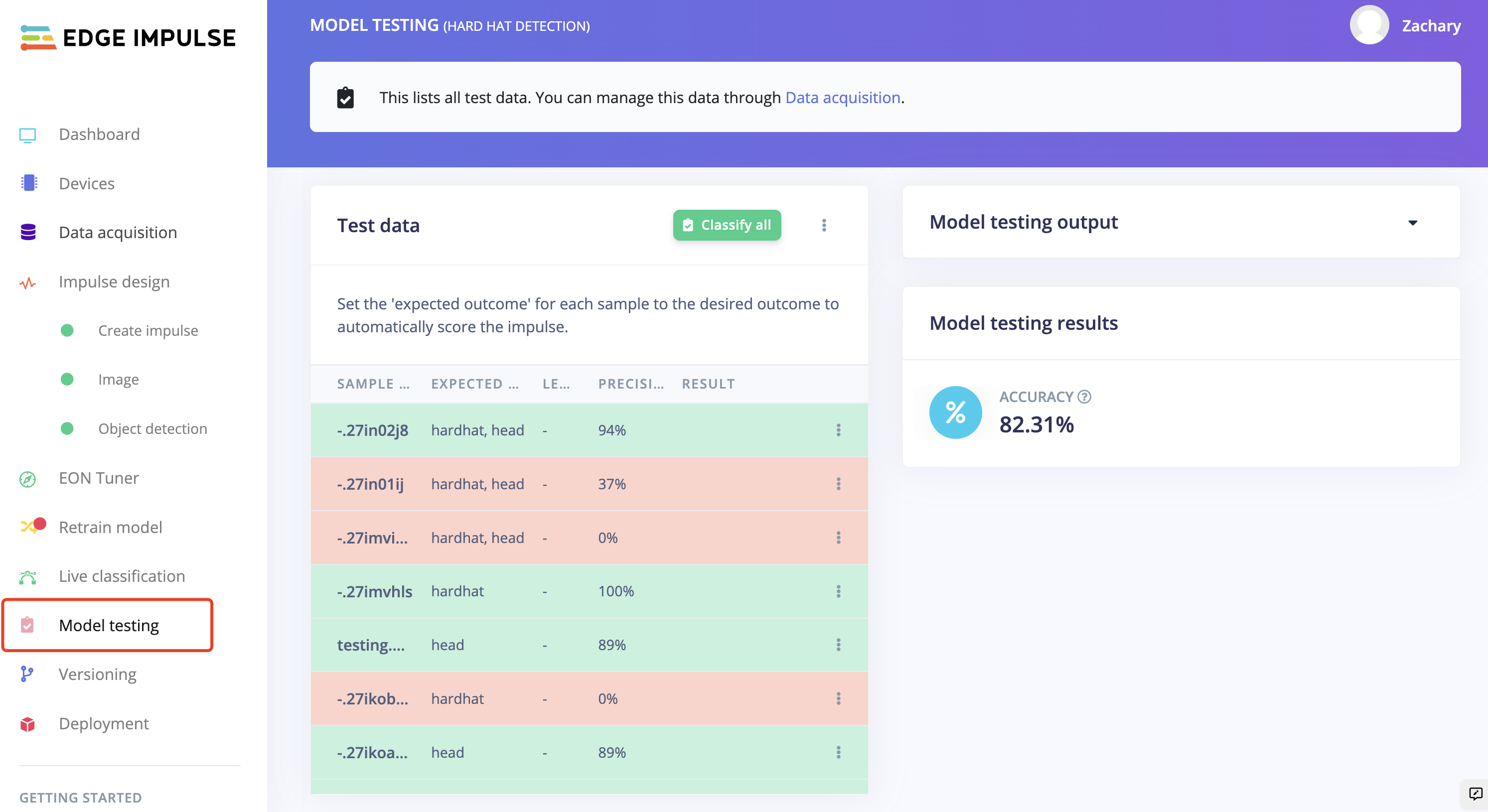
基于定制化 PC 摄像头数据集的 ML 模型训练
Edge Impulse 提供了几种收集数据的方法。 在这里,我们将定制自己的图像,并通过 PC 摄像头捕捉图片上传到网站。
- 步骤 1. 停留在 "仪表板" 页面,然后点击 "让我们收集一些数据"。
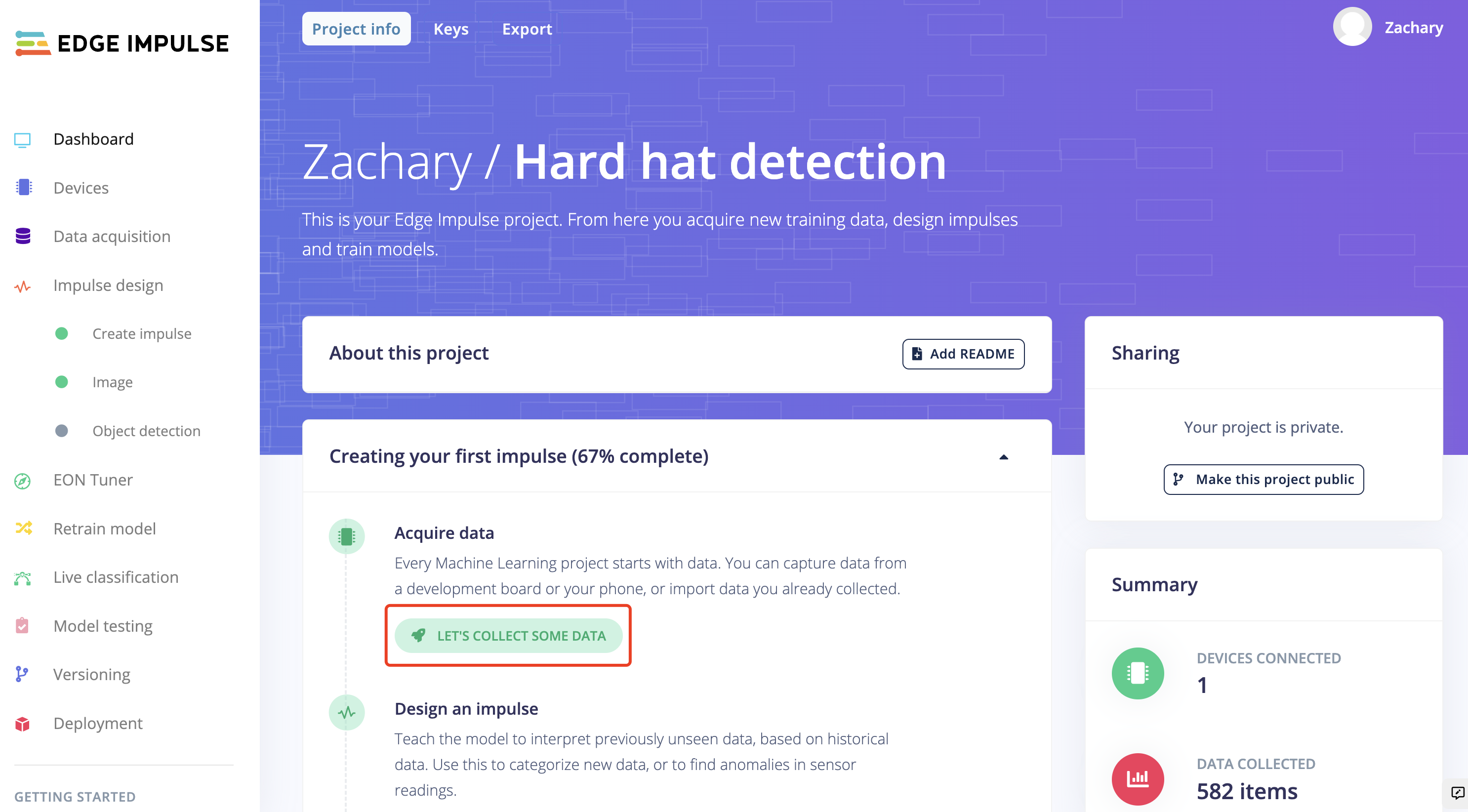
我们可以选择多种选项来收集数据,这里我们使用计算机进行操作。
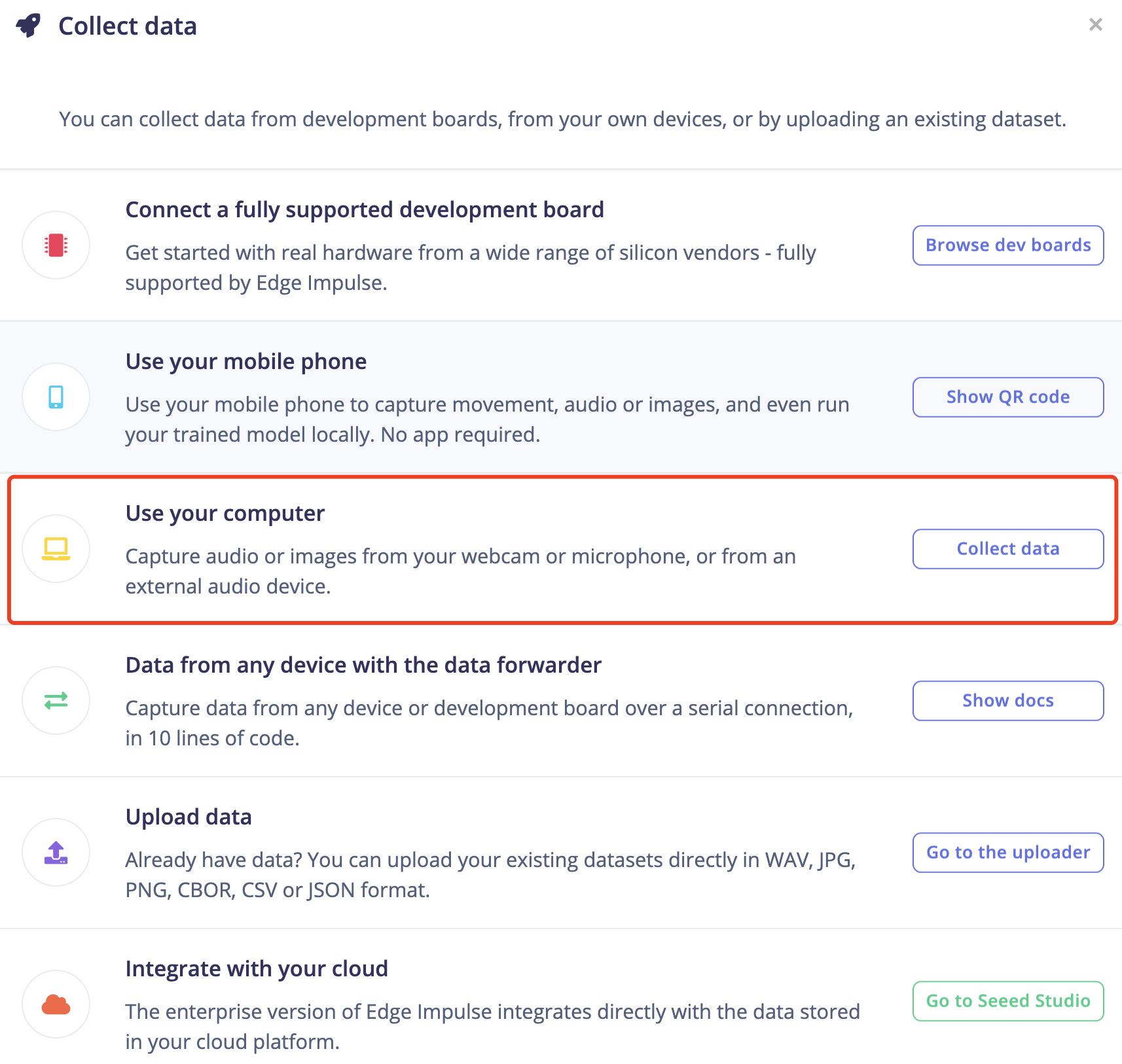
- 步骤 2. 过一会儿,页面将显示已连接到计算机。 点击 "收集图像?" 然后 "授予相机访问权限"。
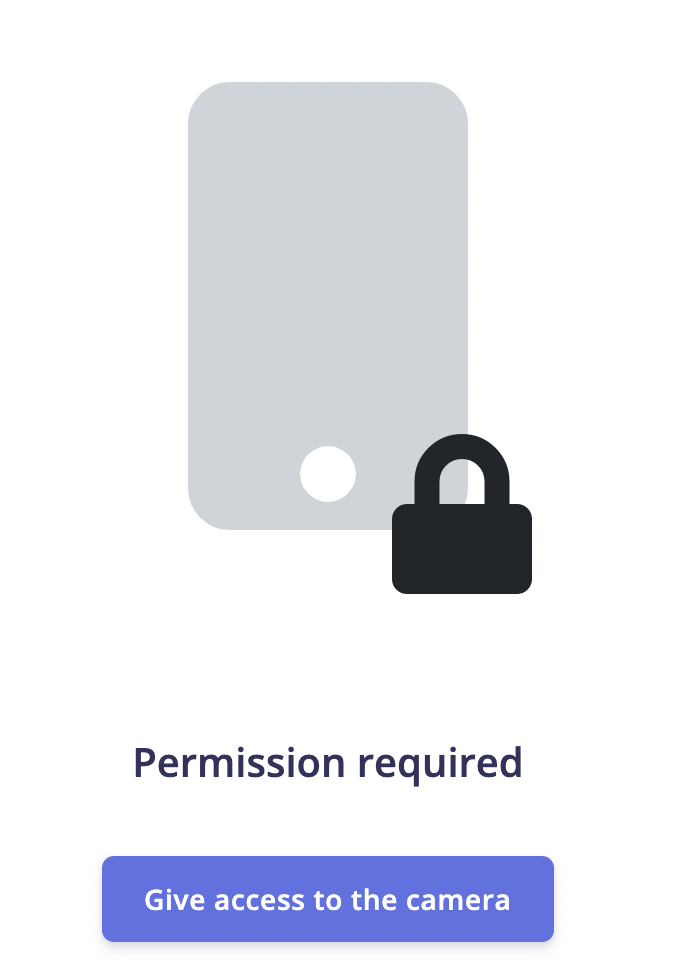
- 步骤 3. 点击 "捕捉" 来拍摄你自己或其他人的照片。 图像数据需要标记为"安全帽"和"头部"。 为了快速标记图片,强烈建议在转到下一个类别之前完成一个类别的数据采集,即建议先完成"安全帽"的图片采集,然后再转到"头部"的图片采集。
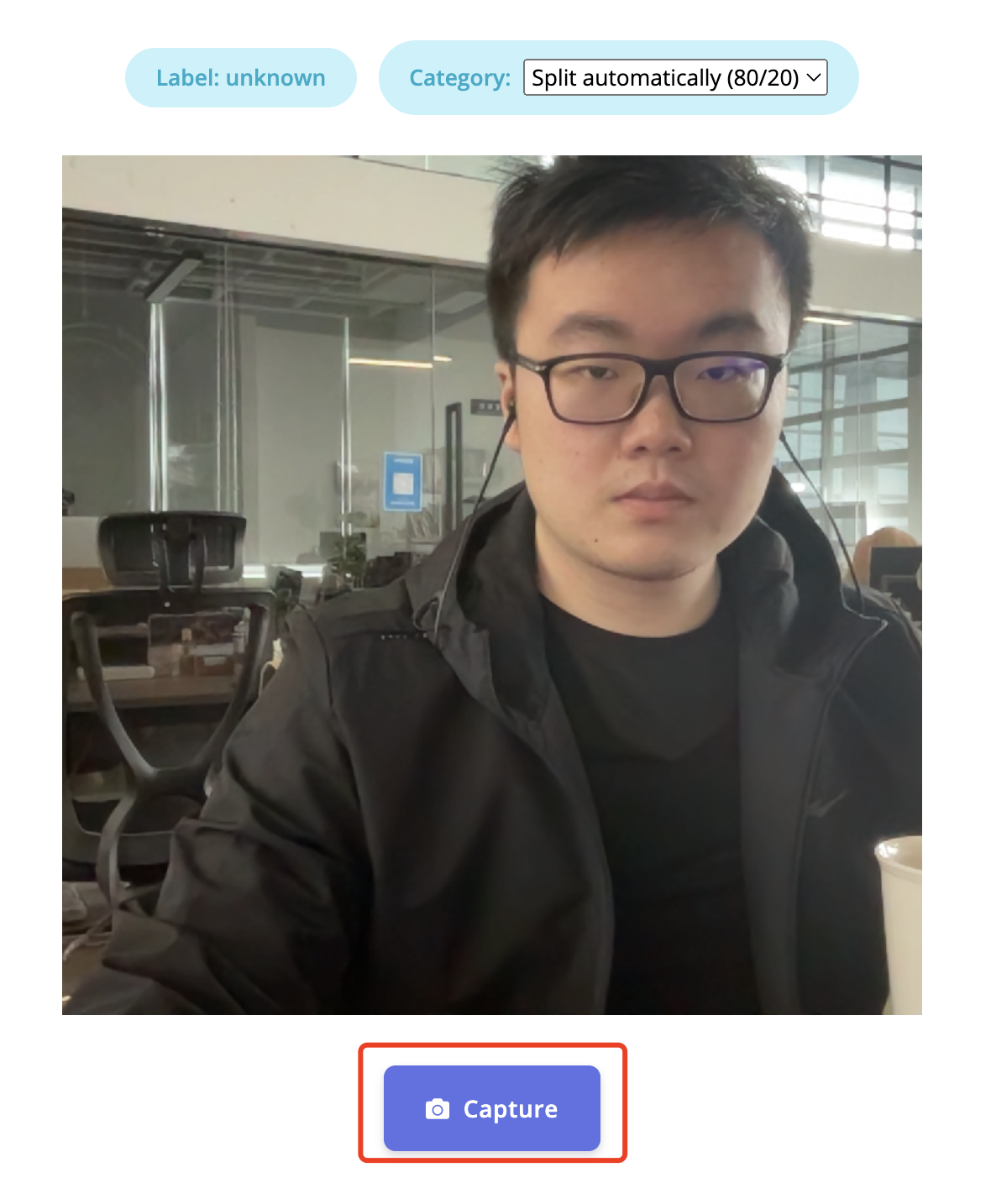
捕获的图片将自动存储在"数据采集"中。 为了提高训练模型的性能,强烈建议尽可能多地收集图片,并在不同类别中收集相同数量的数据。
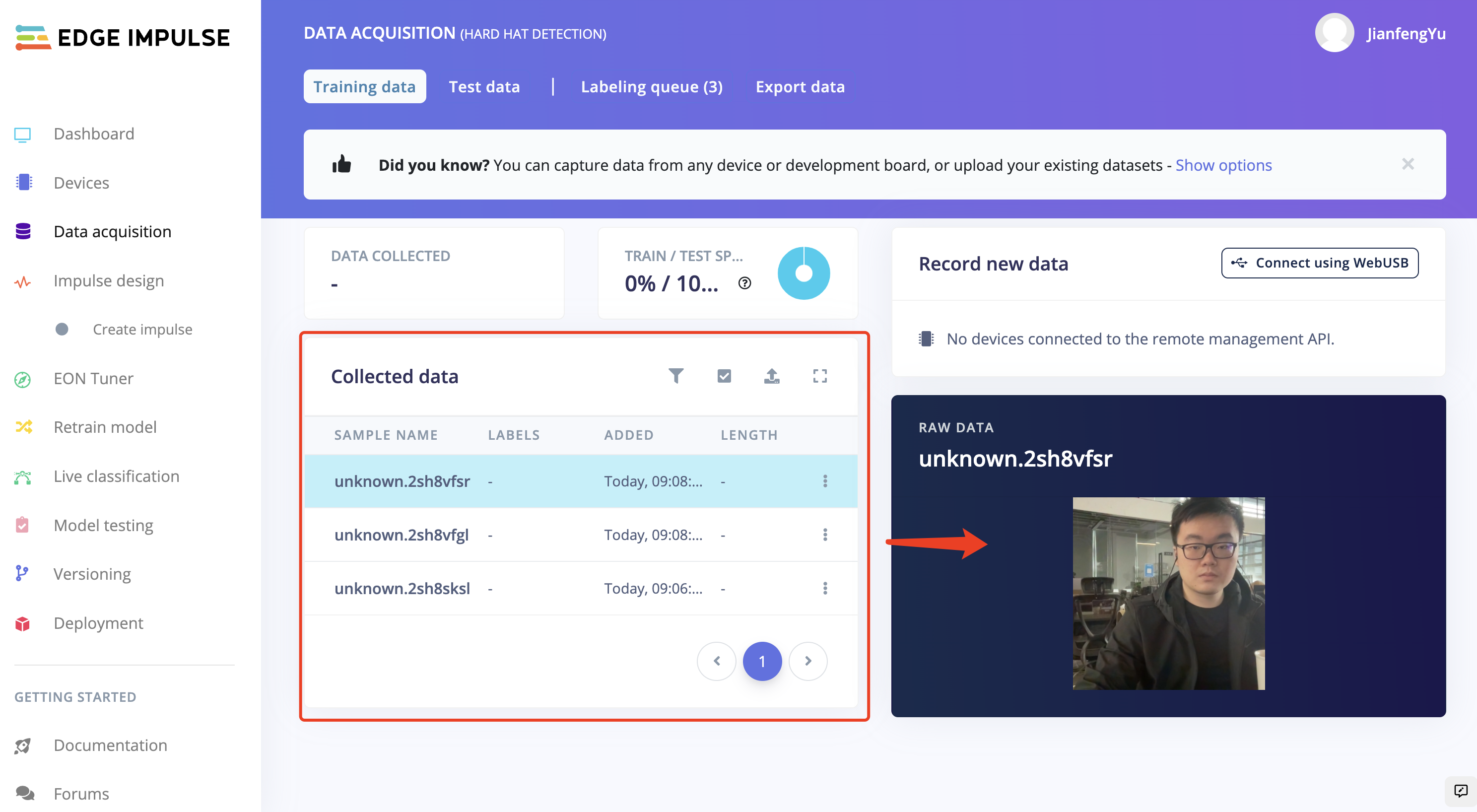
- 步骤 4. 点击"标记队列"通过在图片上用方框圈出头部来标记数据。
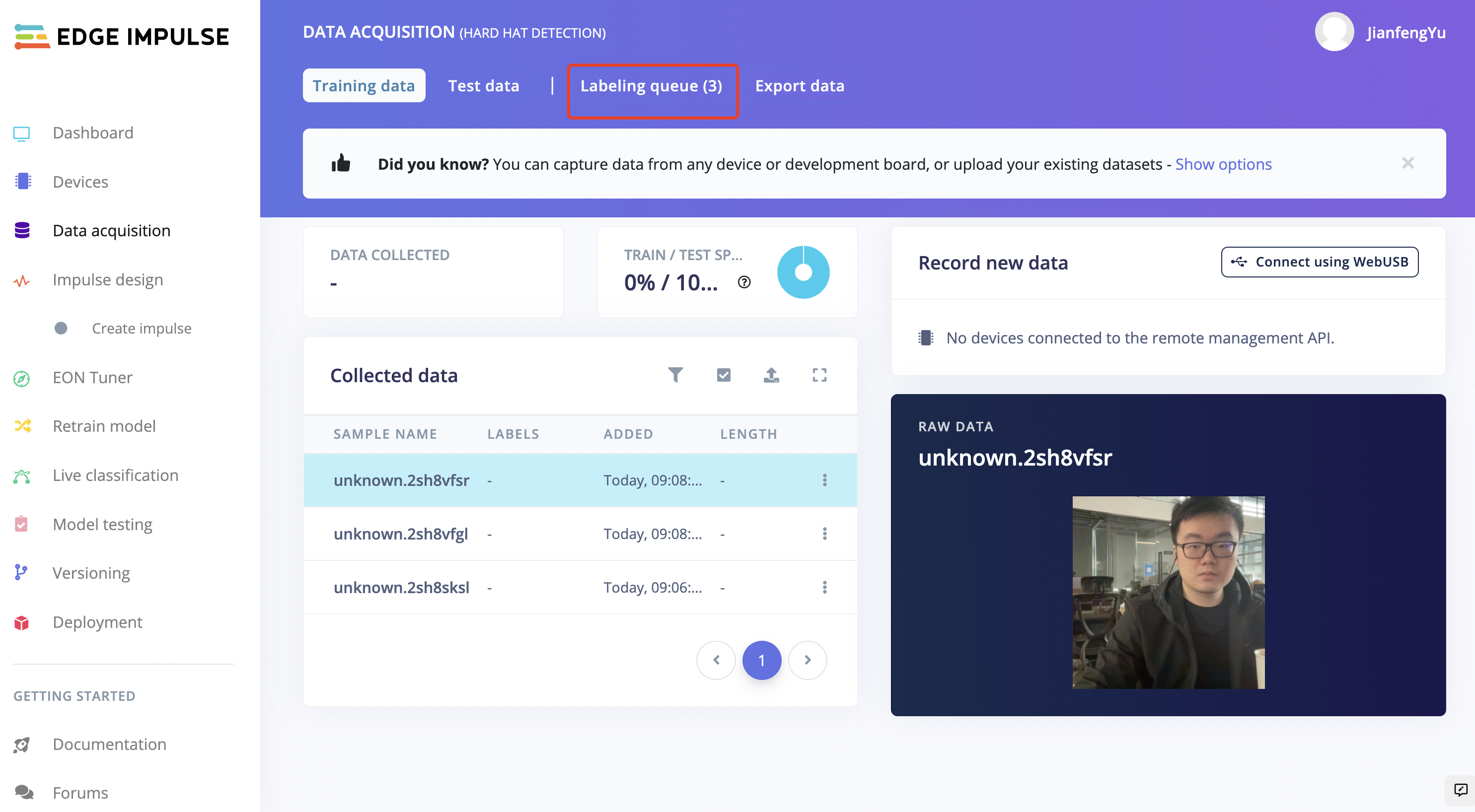
使用鼠标拖动一个框围绕对象以添加标签。 然后点击保存标签以继续下一个项目。
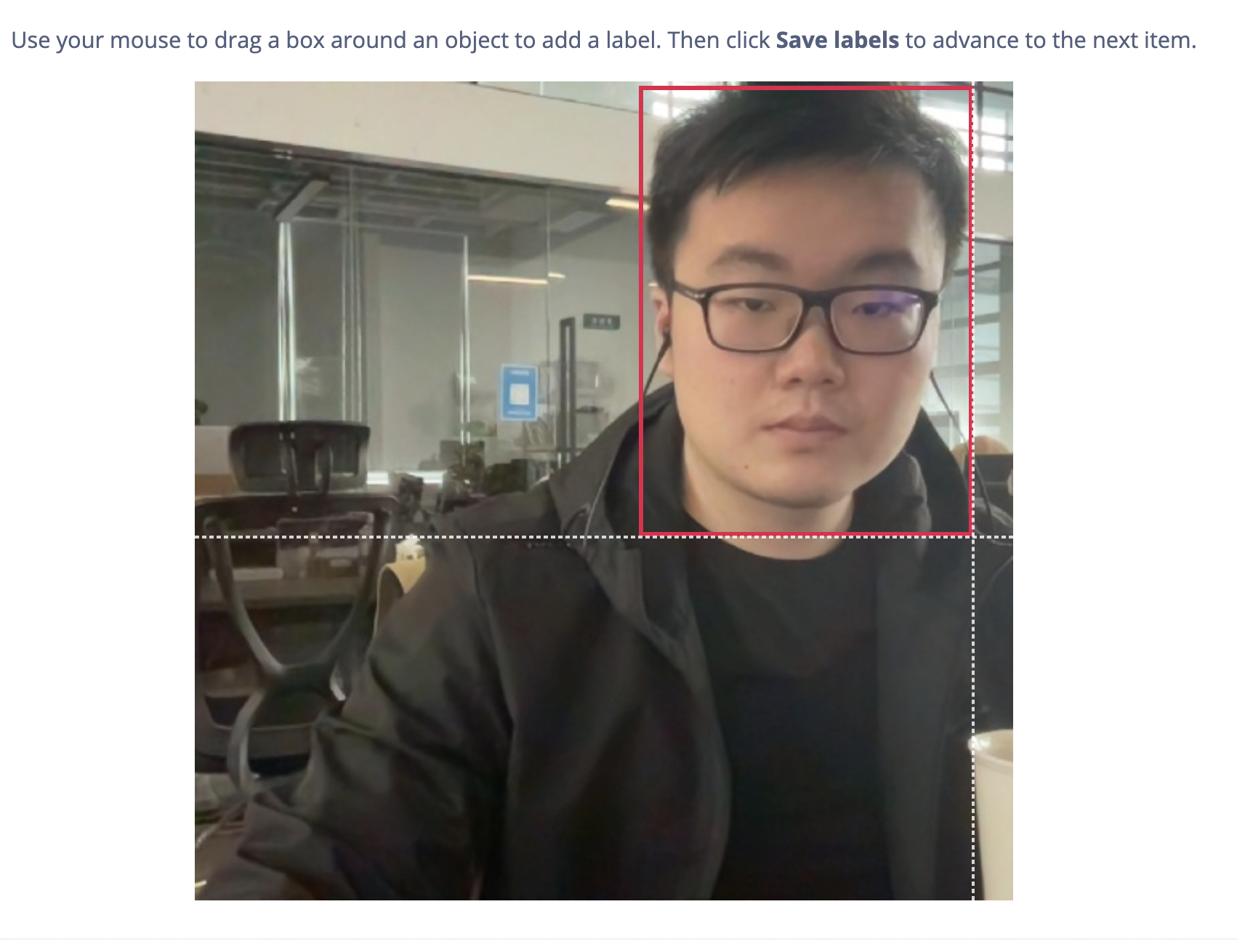
将标签设置为"头部"和"安全帽"并填写对话框。 请确保方框框住人的头部区域。
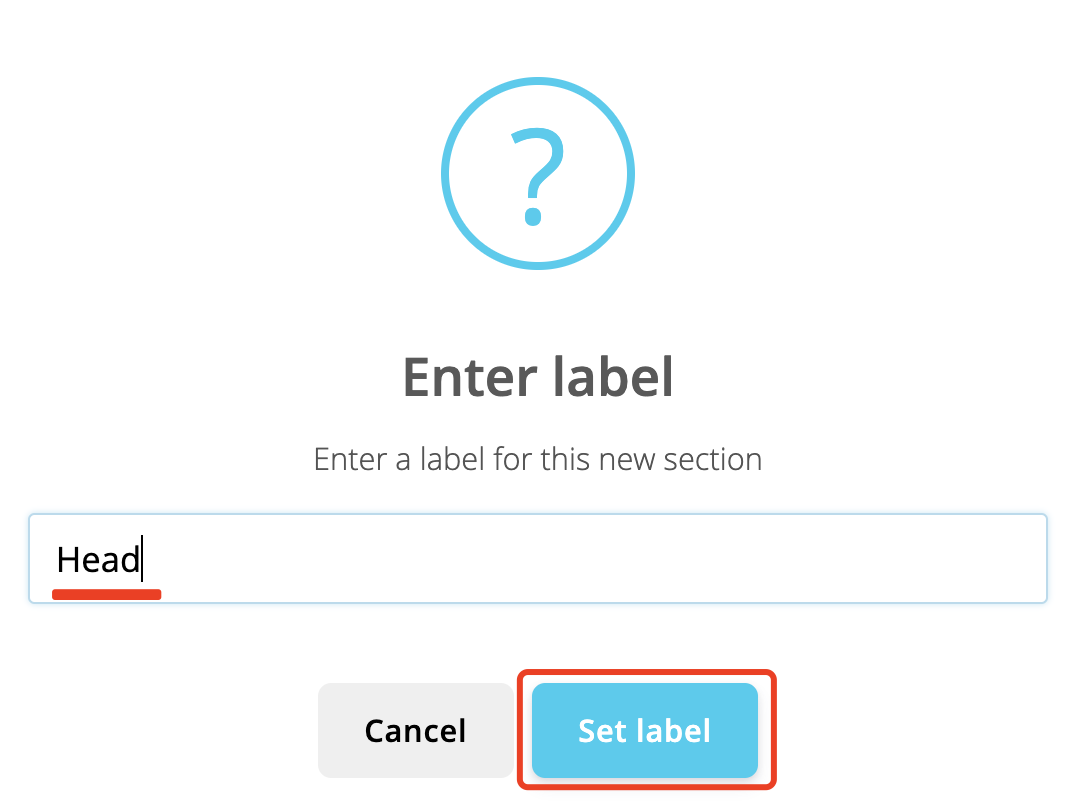
- 步骤 5. 当数据被标记后,点击"保存标签"并移动到"冲动设计"
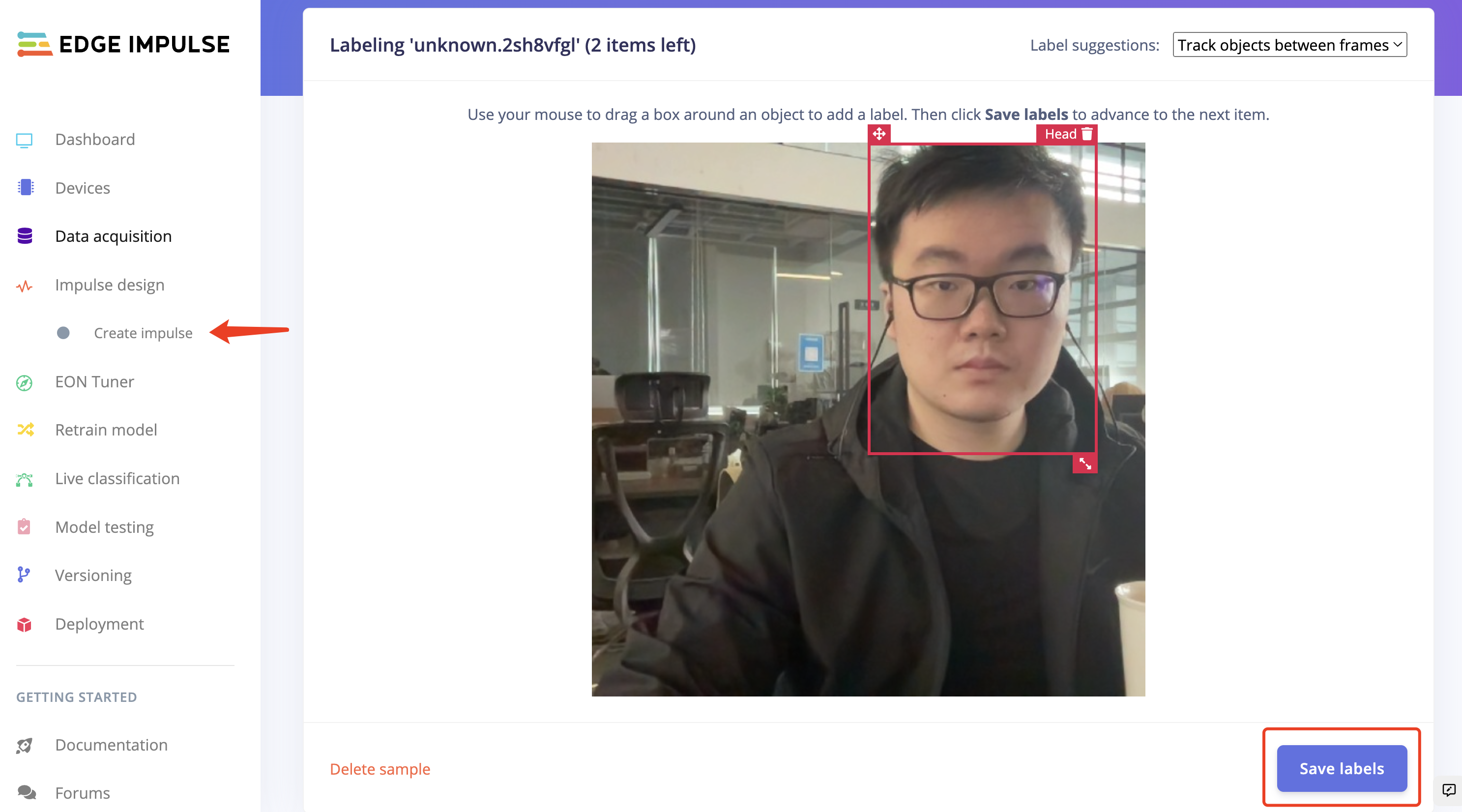
- 步骤 6. 选择合适的图像处理块和图像学习块并保存冲动。
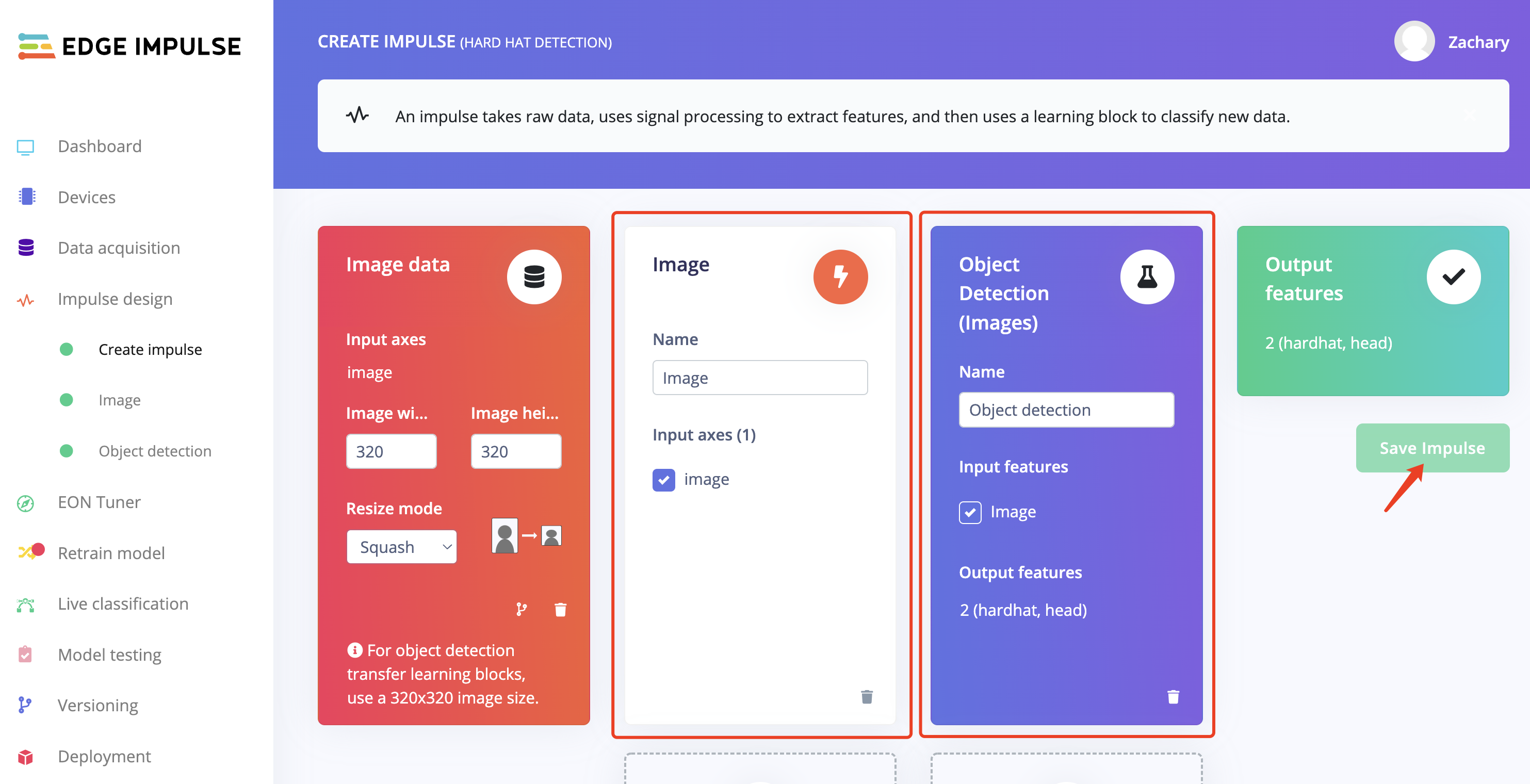
- 步骤 7. 点击页面左侧的"图像"。
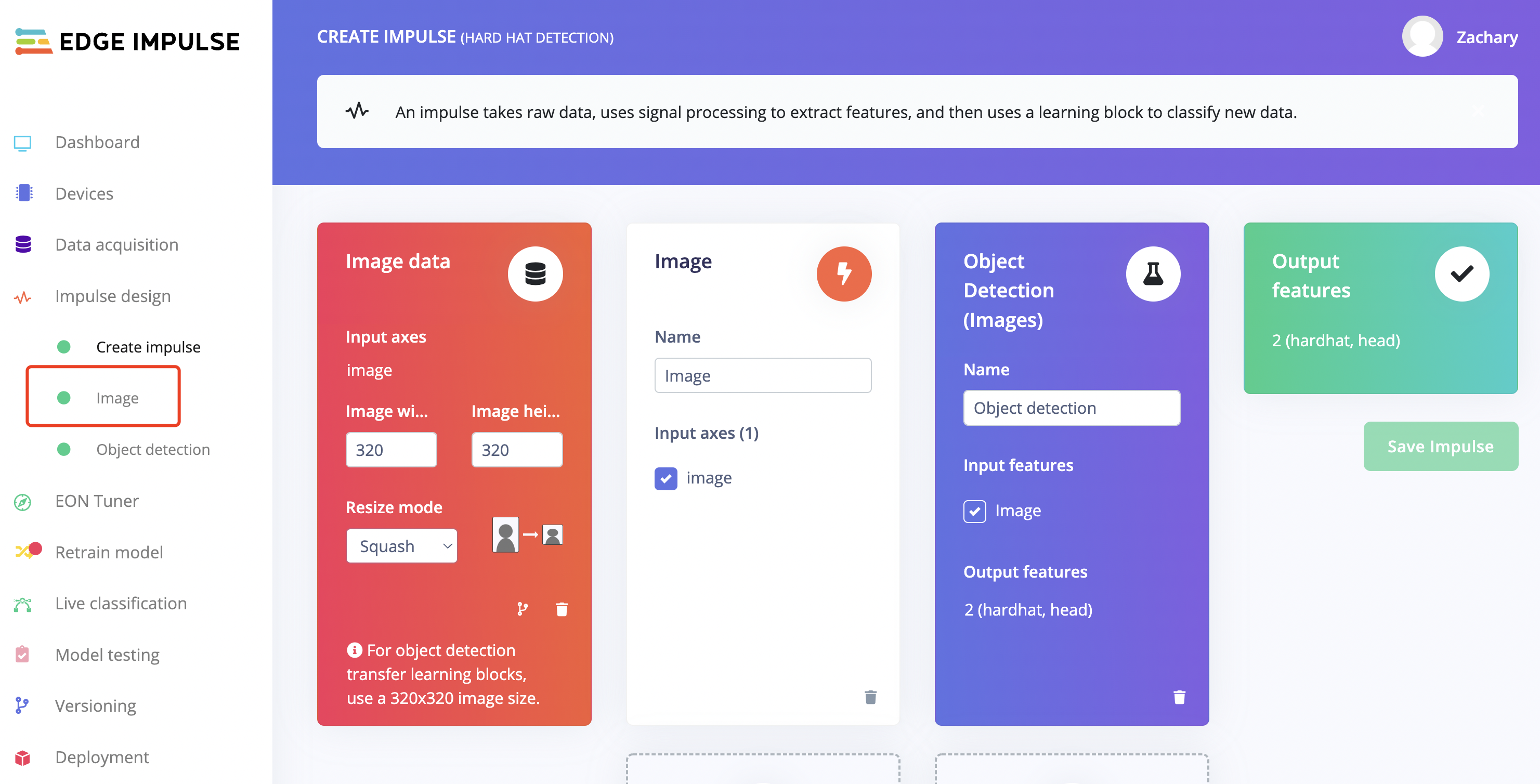
配置为"GRB"并点击"保存参数",页面将自动转到"生成特征"网站。
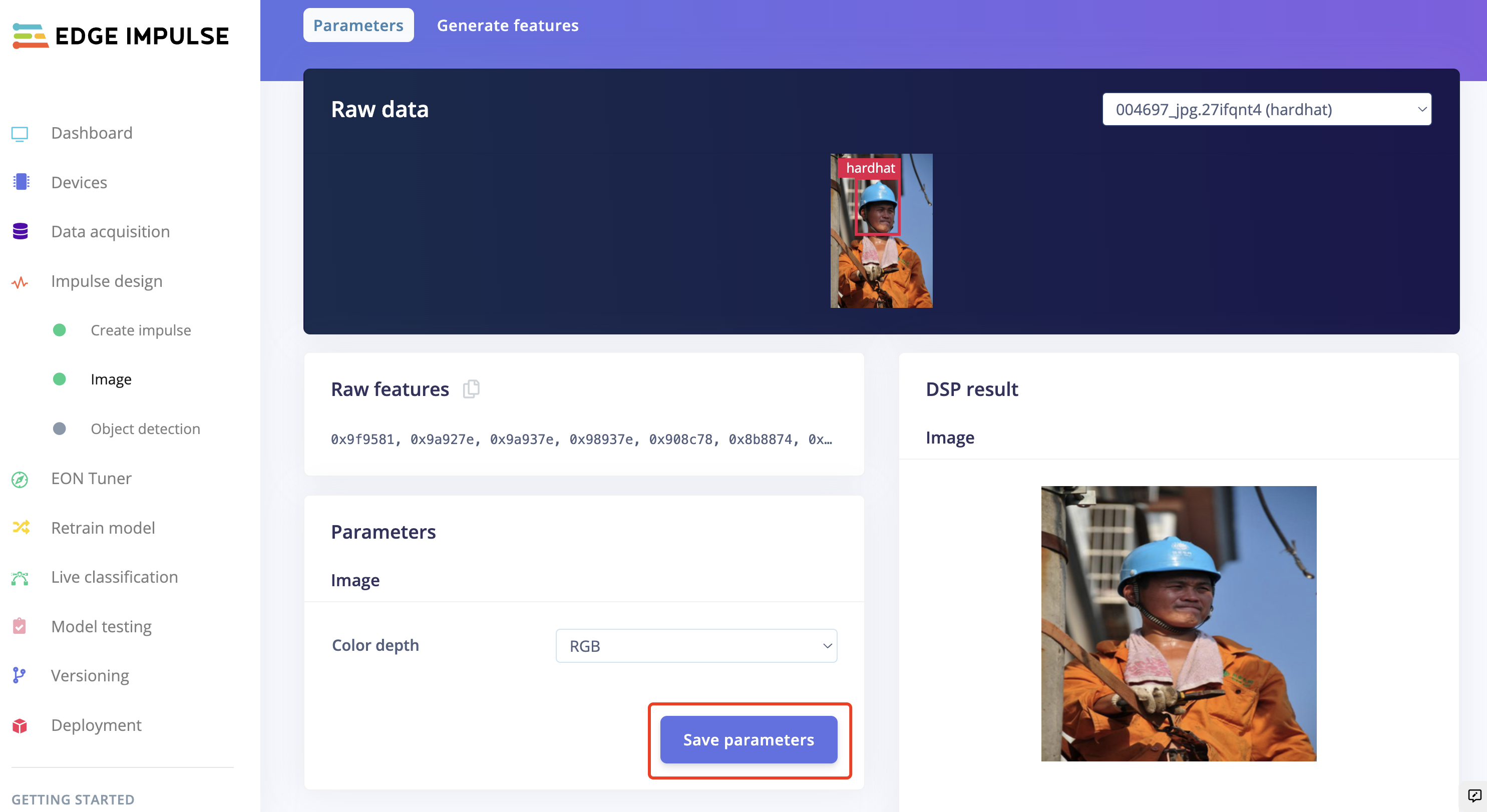
我们能够生成特征。
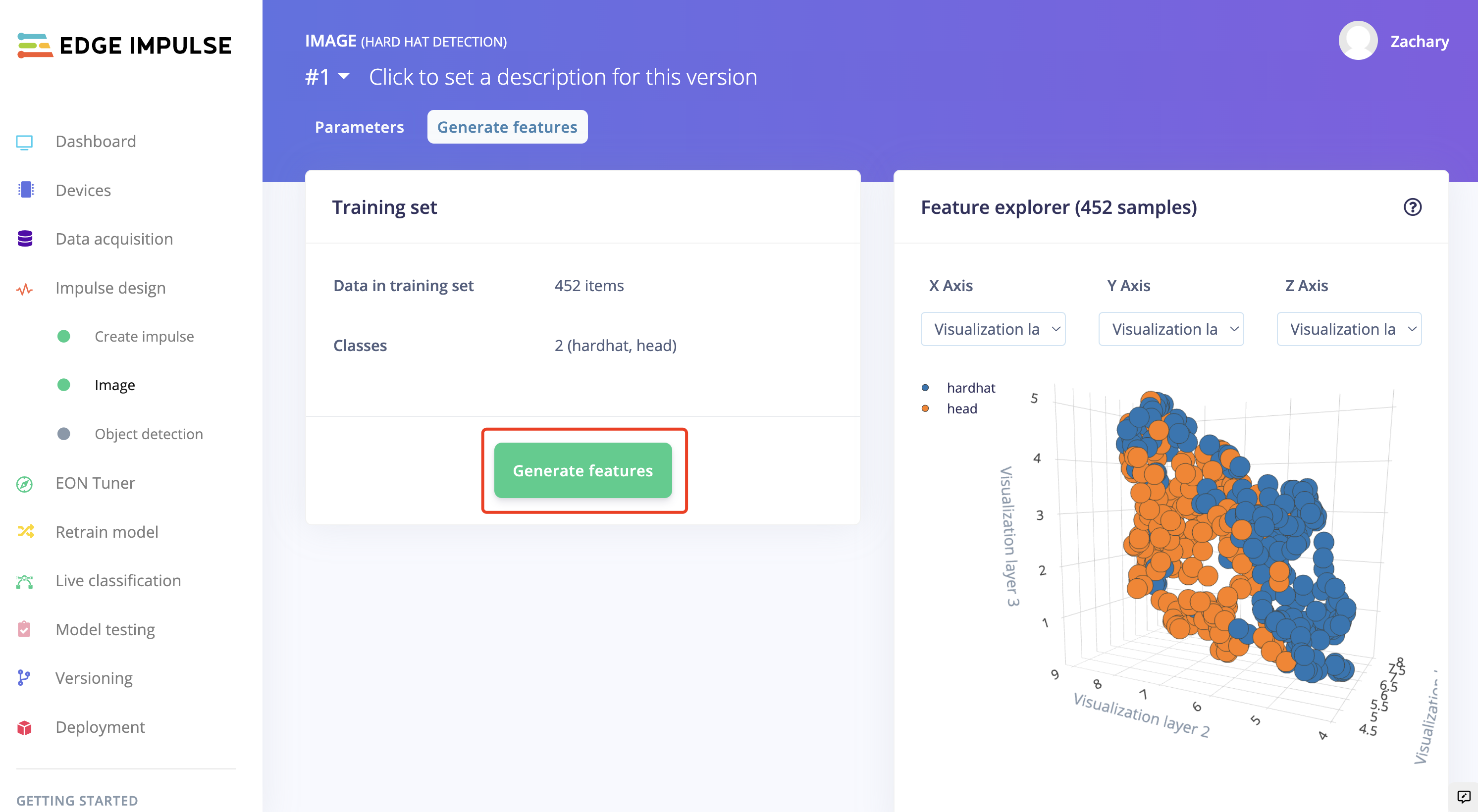
- 步骤 8. 当出现 "任务完成" 时,点击页面左侧的 "目标检测"。
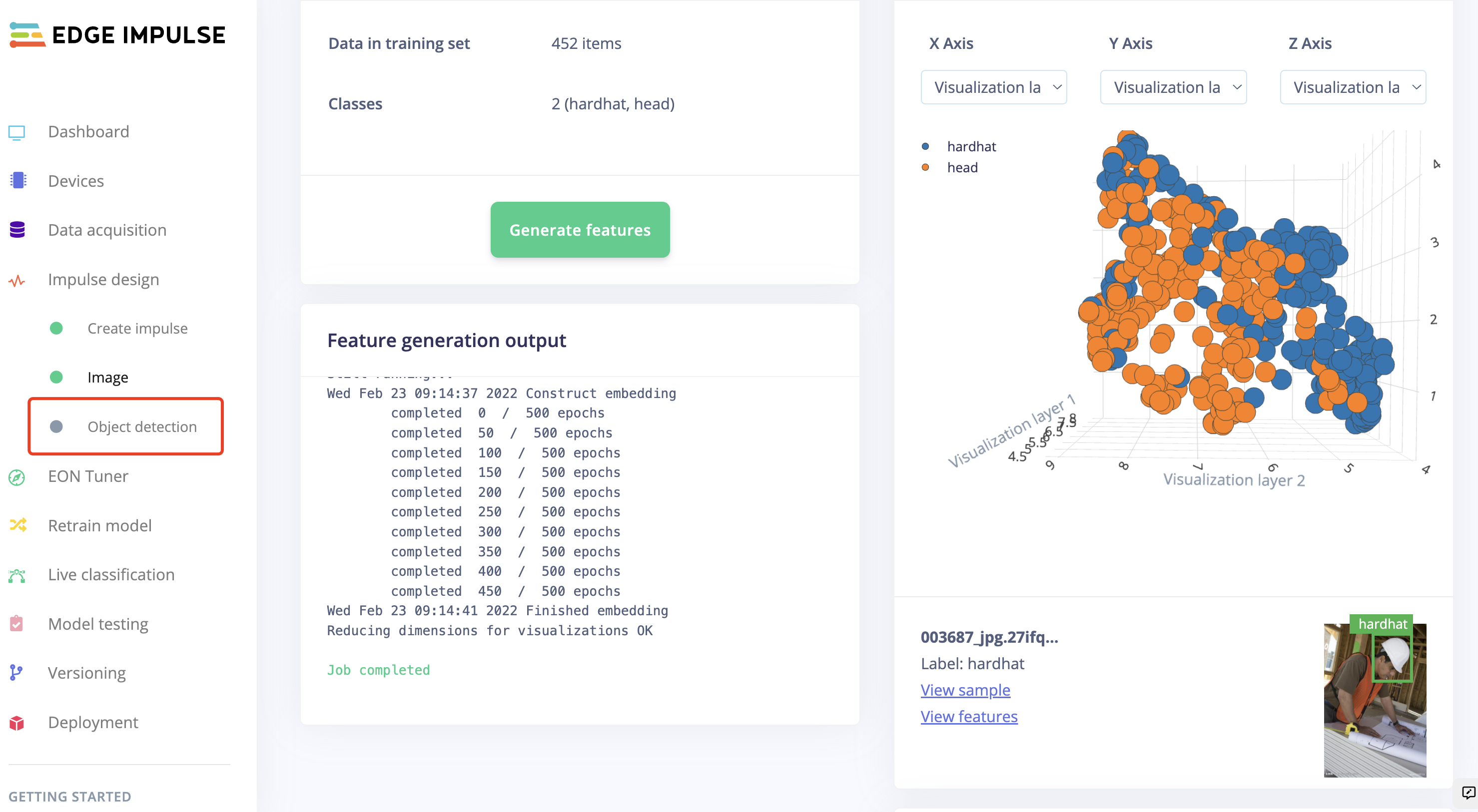
点击 "开始训练",让 Edge Impulse 根据生成的特征训练模型。
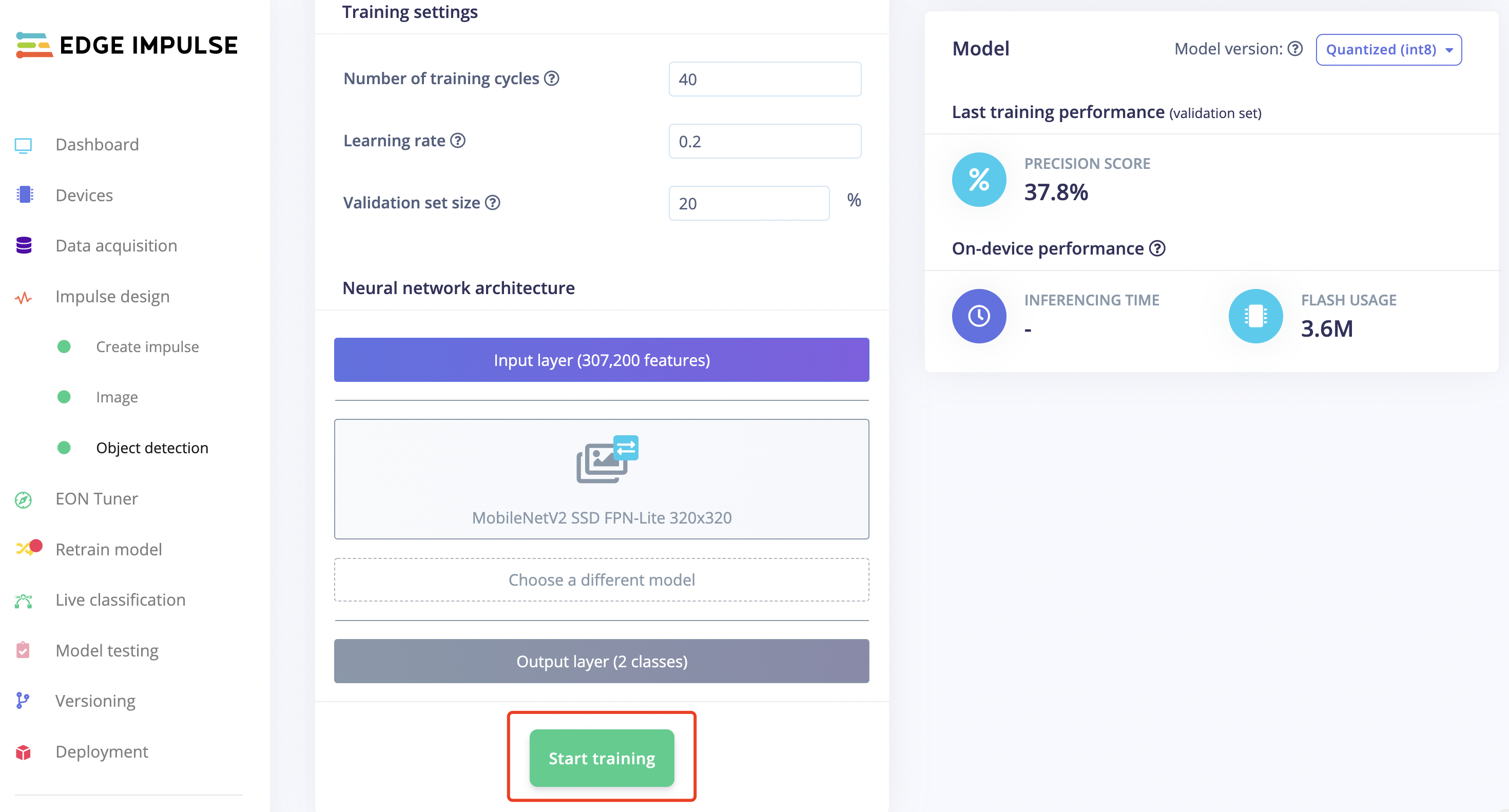
- 步骤 9. 一旦出现 "任务完成",点击 "模型测试" 来检查模型的工作情况。
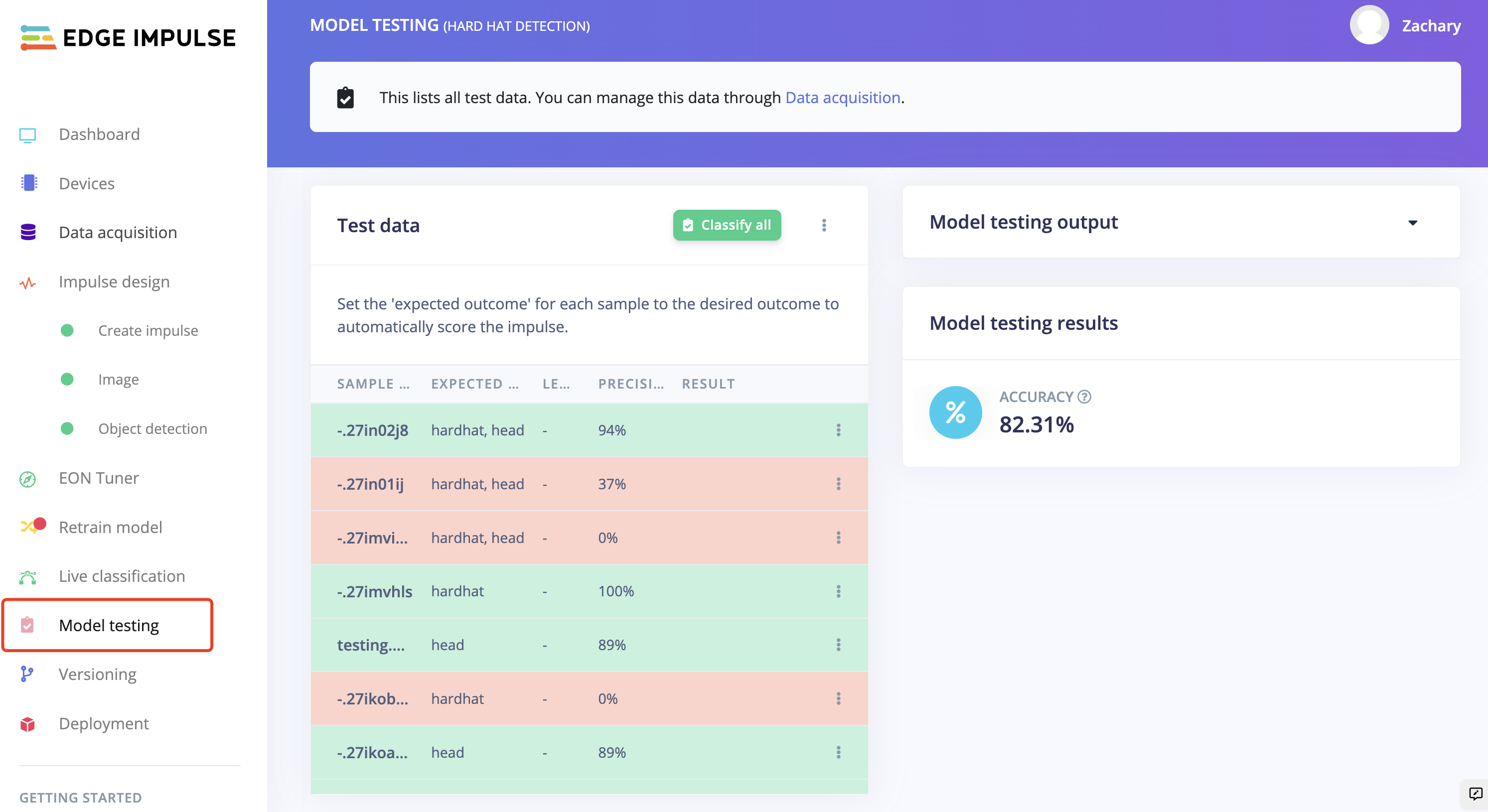
基于定制的NVIDIA Jetson相机数据集的机器学习模型训练
Edge Impulse 提供了几种收集数据的方法。 在这里,我们将定制自己的图像,并通过连接到NVIDIA Jetson Nano的相机捕捉图片上传到网站。
- 步骤 1. 根据硬件进行设置 NVIDIA Jetson Nano 开发套件 或 NVIDIA Jetson Nano 2GB 开发套件 用于连接外部显示器和键盘。 将显示屏连接到 Jetson Nano。
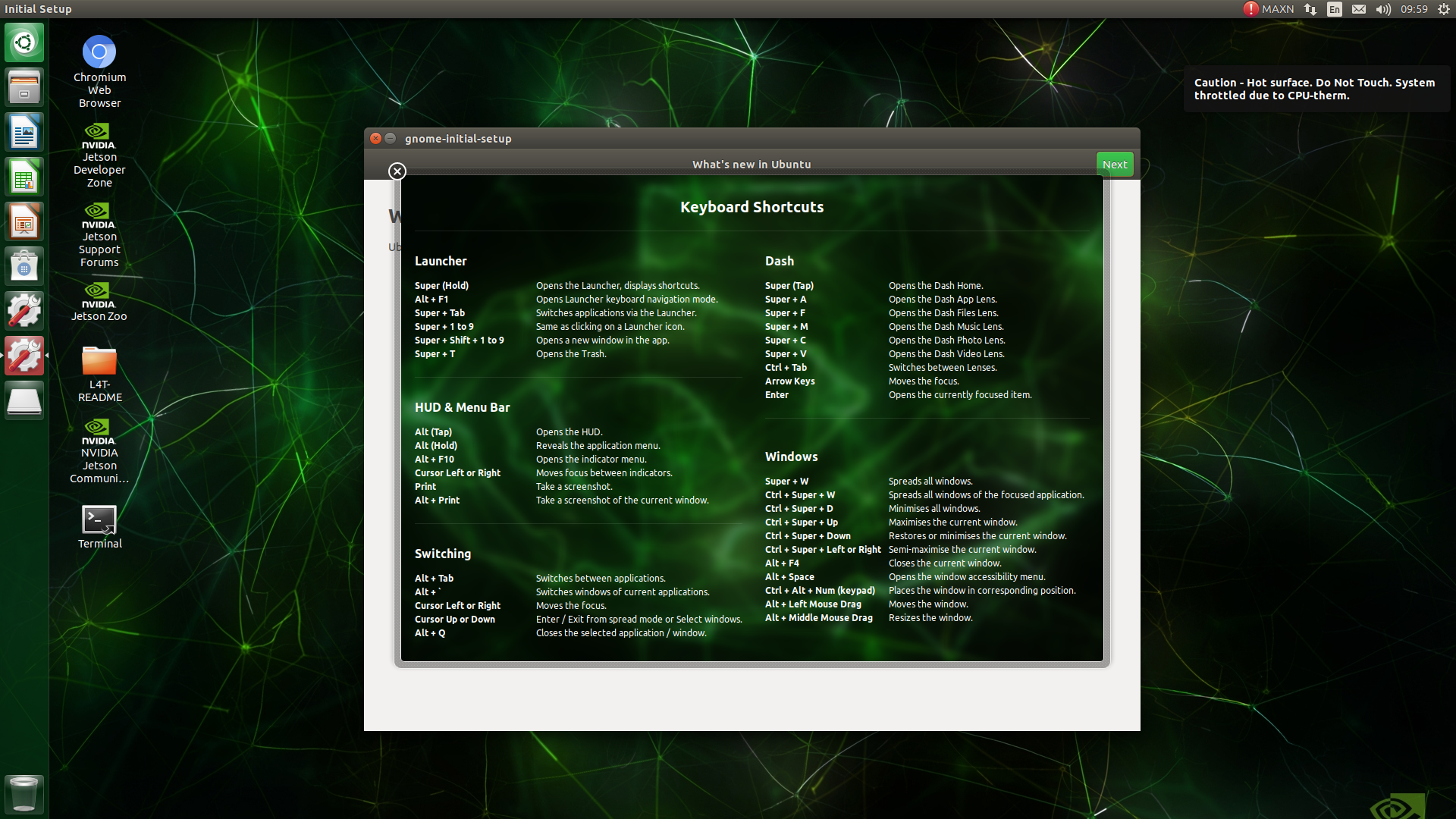
- 步骤 2. 确保您的 Jetson Nano 已连接到互联网,并在 Edge Impulse 中设置设备。
您可以使用以下命令检查网络:
ping -c 3 www.google.com
如果网络正常,结果应如下所示:
3 packets transmitted, 3 received, 0% packet loss, time 2003ms
设置开始运行,使用以下命令:
edge-impulse-linux
然后网站将请求 Edge Impulse 账户。
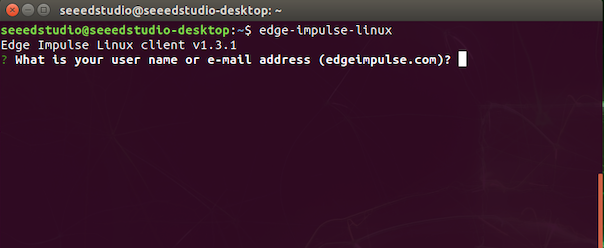
如下所示的内容意味着连接已完成。 我们在 Edge Impulse 中保存的所有项目都是可选择的。
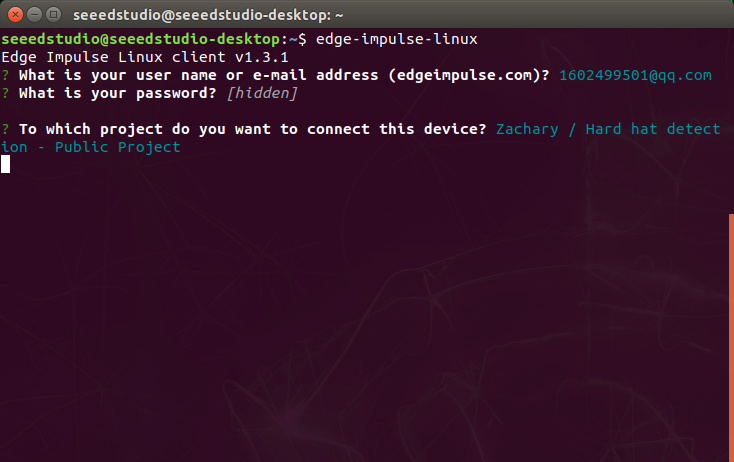
我们正在捕捉照片,因此这里需要选择我们的 USB 摄像头以在网站上应用。
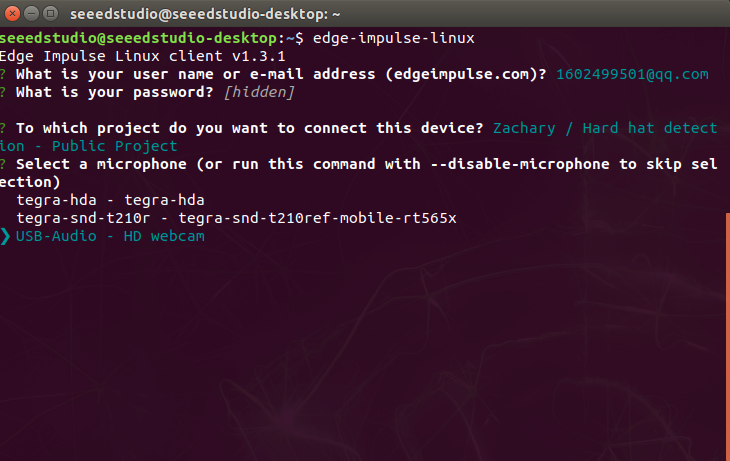
命名我们想要连接到网站的设备
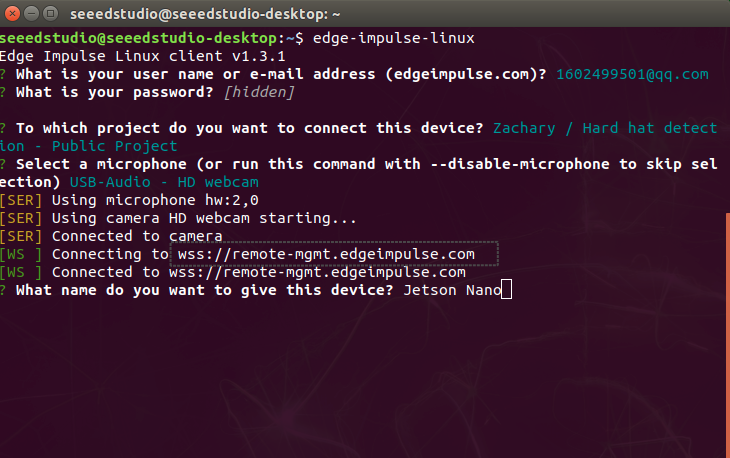
很明显,设备 Jetson Nano 现在已连接到项目。
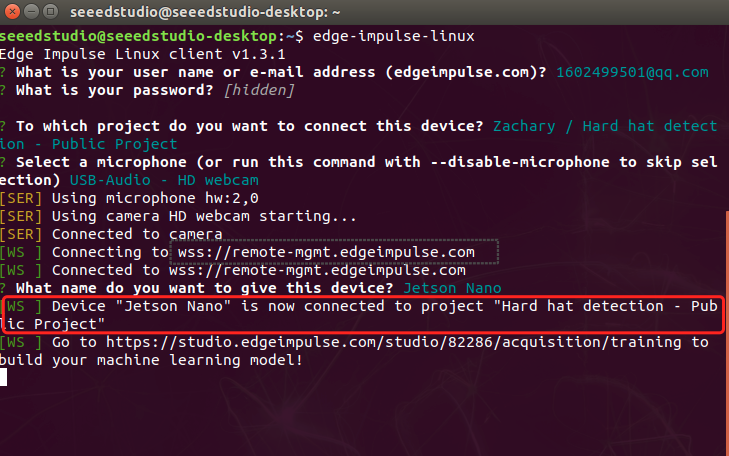
- 步骤 3. 返回 Edge Impulse 页面并选择 "设备" 列。 连接的 Jetson Nano 如下所示:
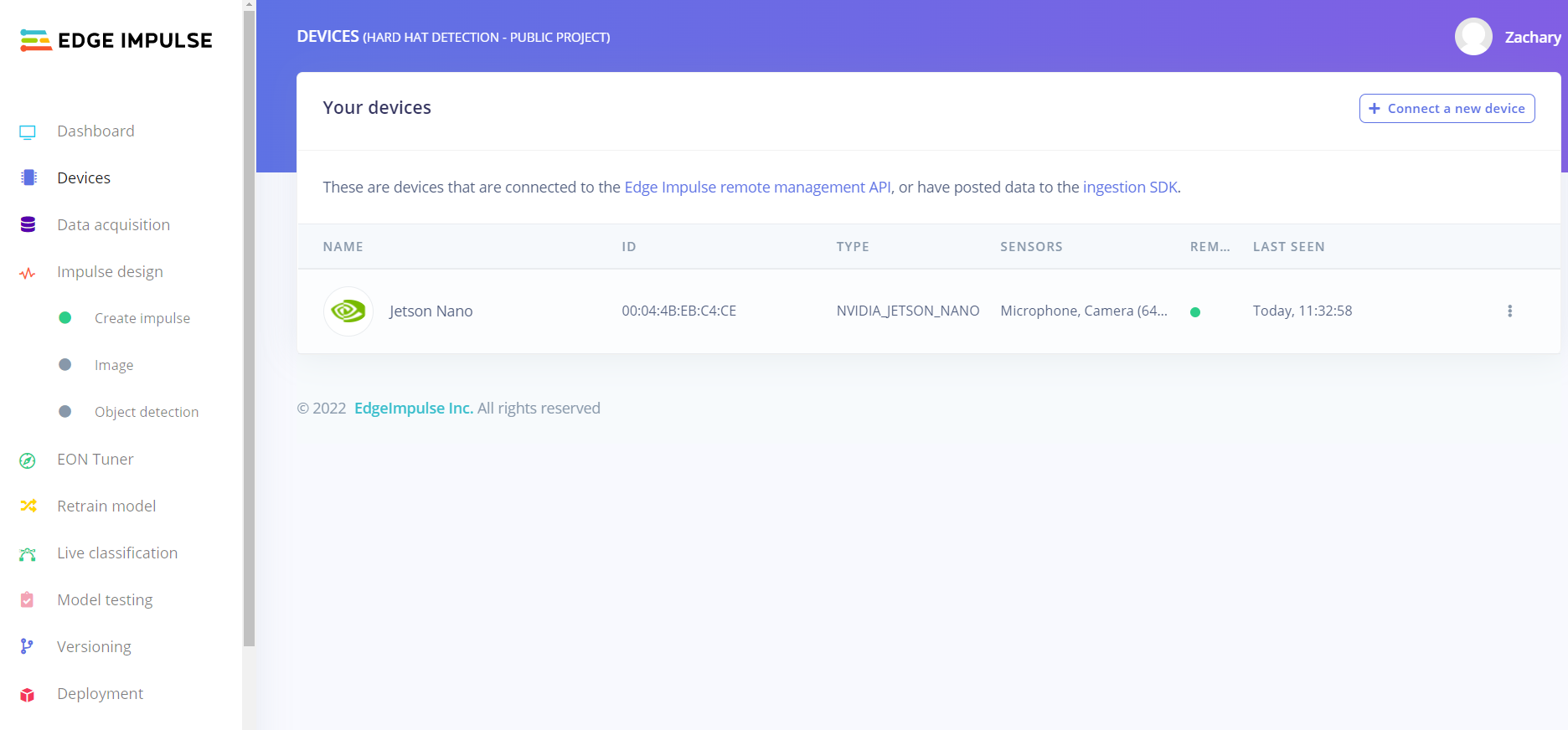
- 步骤 4. 选择我们连接到 Edge Impulse 的设备,然后转到 "数据采集" 页面。 点击 "捕捉" 来拍摄你自己或其他人的照片。 图像数据需要标记为"安全帽"和"头部"。 为了快速标记图片,强烈建议在转到下一个类别之前完成一个类别的数据采集,即建议先完成"安全帽"的图片采集,然后再转到"头部"的图片采集。
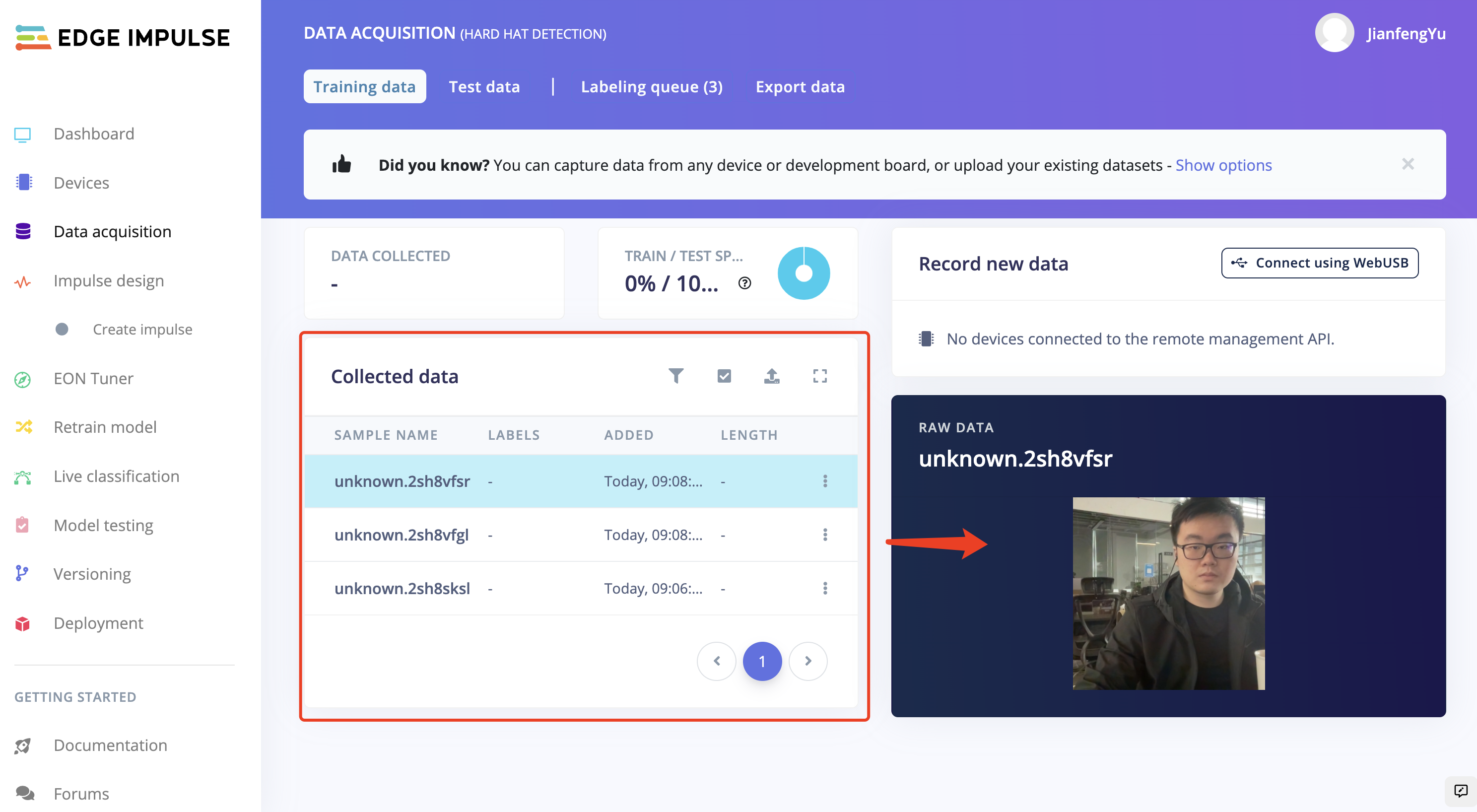
捕获的图片将自动存储在"数据采集"中。 为了提高训练模型的性能,强烈建议尽可能多地收集图片,并在不同类别中收集相同数量的数据。
步骤 5. 当数据收集完成后,转到 "冲击设计"
步骤 6. 选择合适的图像处理块和图像学习块并保存冲动。
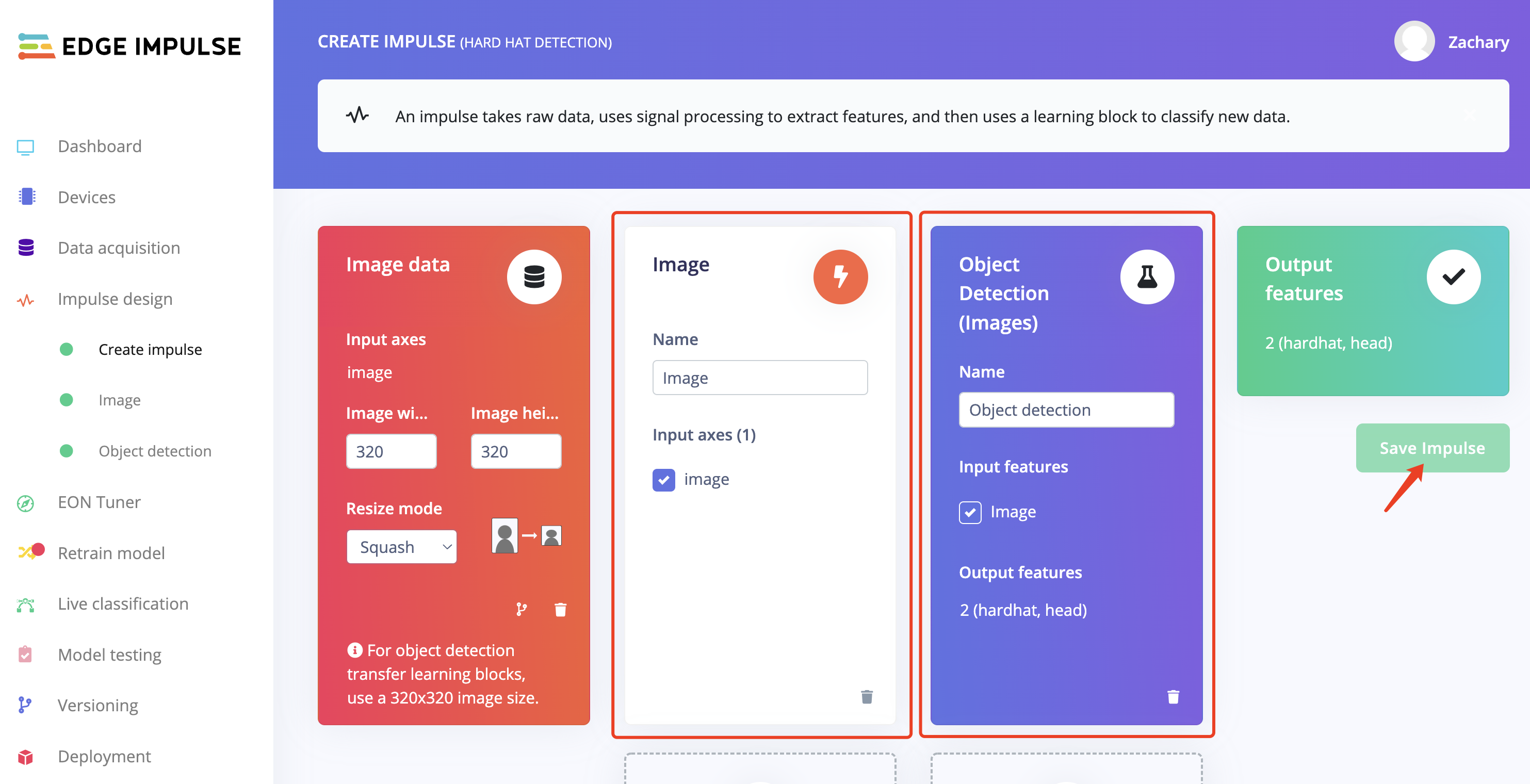
- 步骤 7. 点击页面左侧的"图像"。
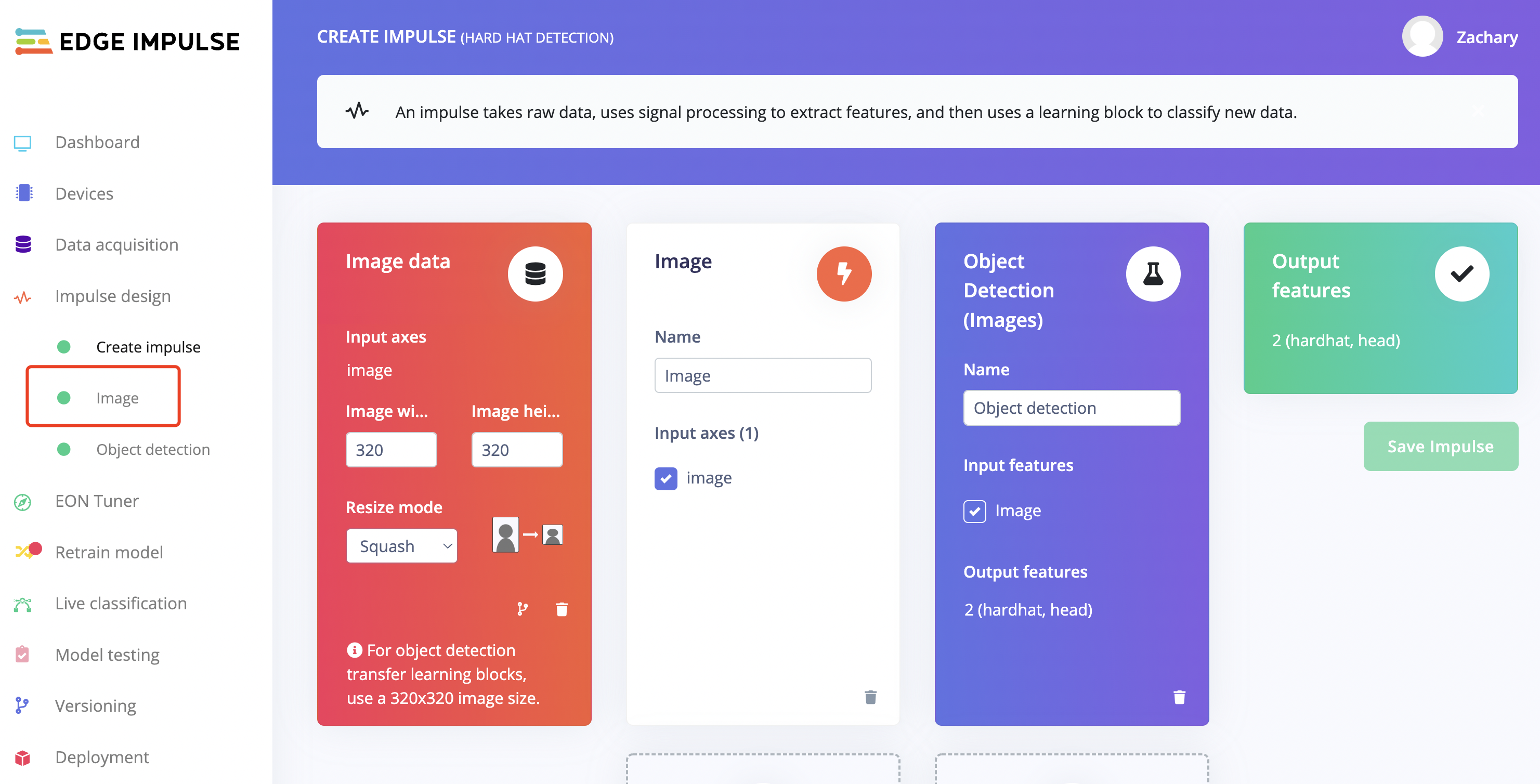
配置为"GRB"并点击"保存参数",页面将自动转到"生成特征"网站。
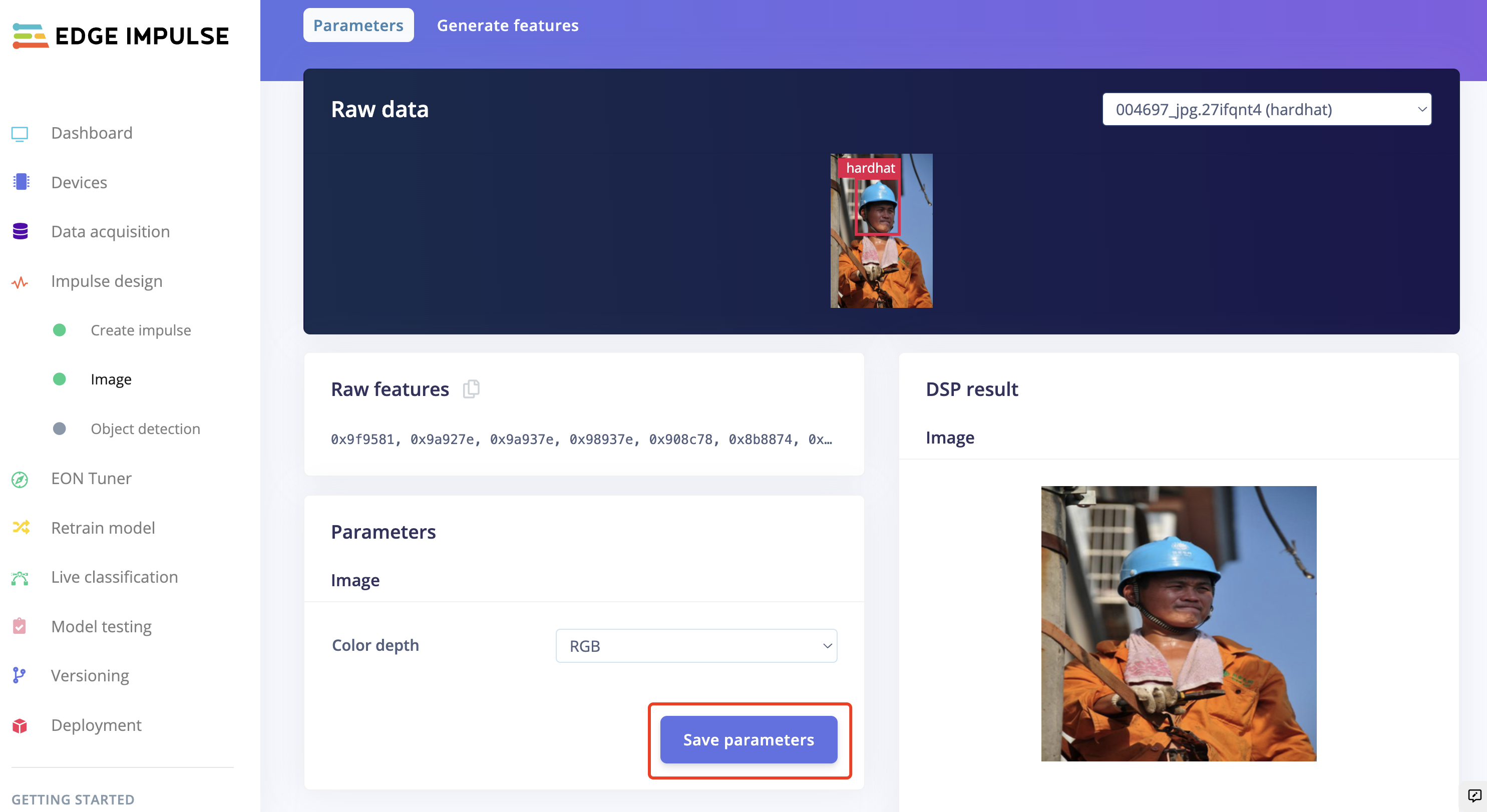
我们能够生成特征。
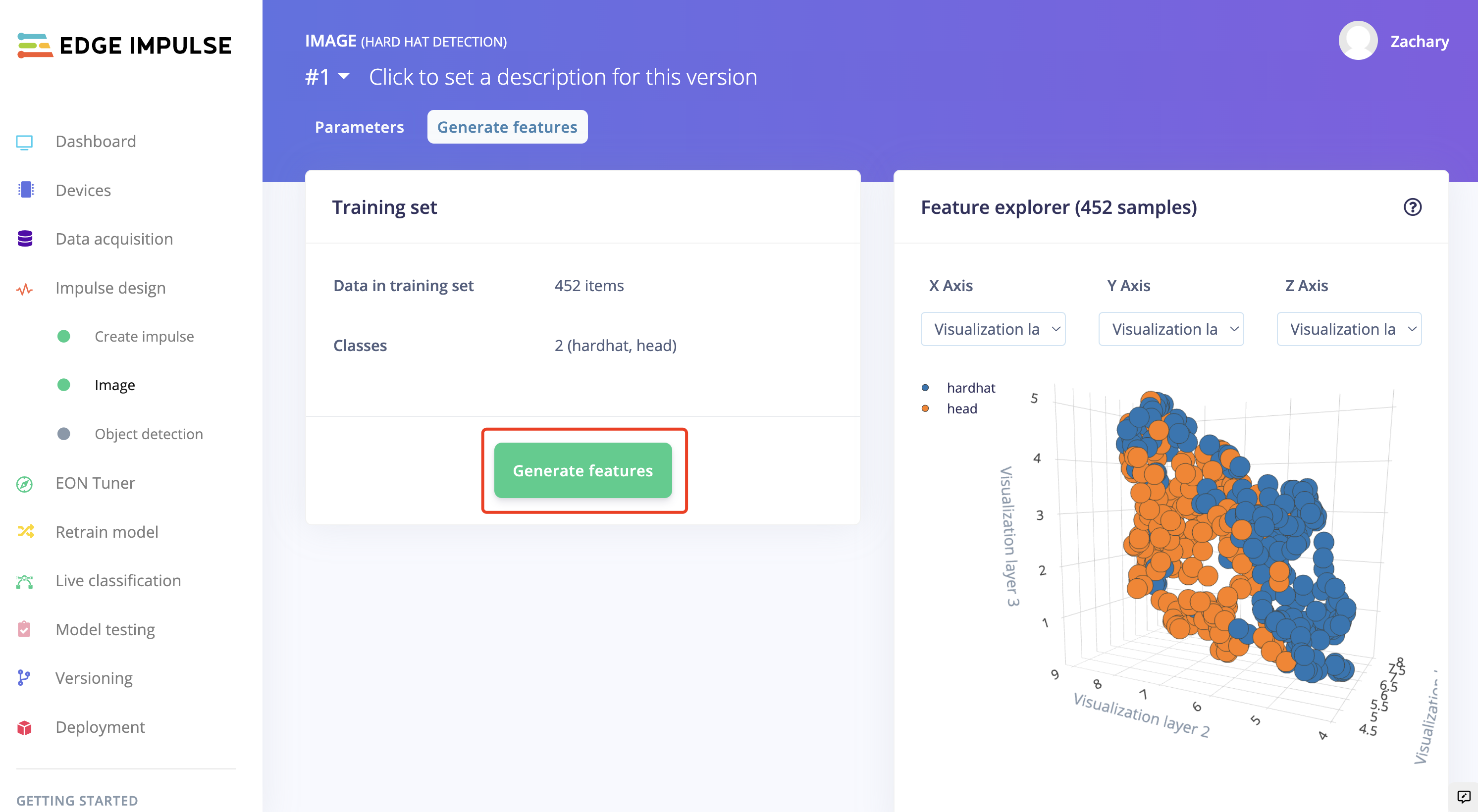
- 步骤 8. 当出现 "任务完成" 时,点击页面左侧的 "目标检测"。
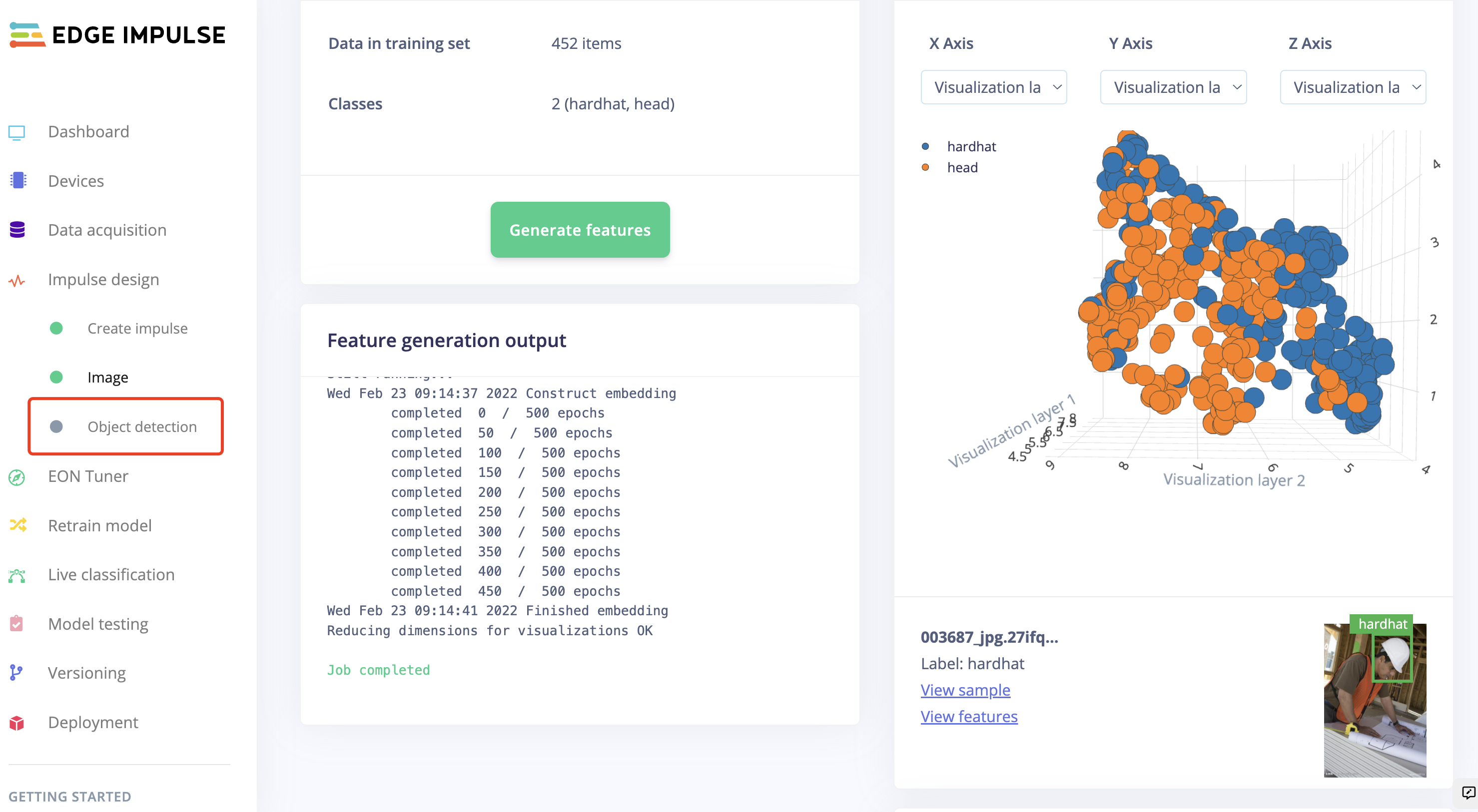
点击 "开始训练",让 Edge Impulse 根据生成的特征训练模型。
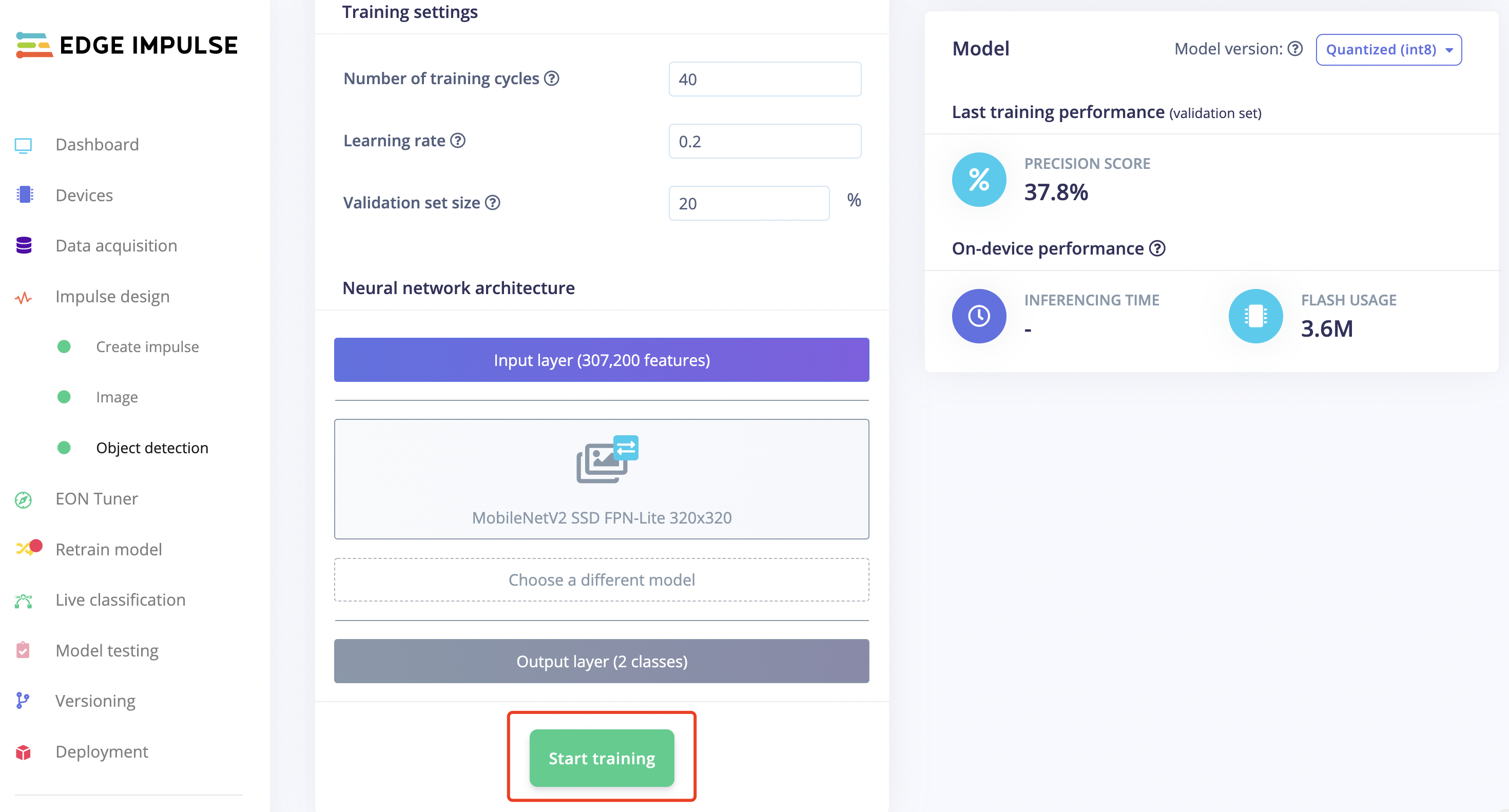
- 步骤 9. 一旦出现 "任务完成",点击 "模型测试" 来检查模型的工作情况。
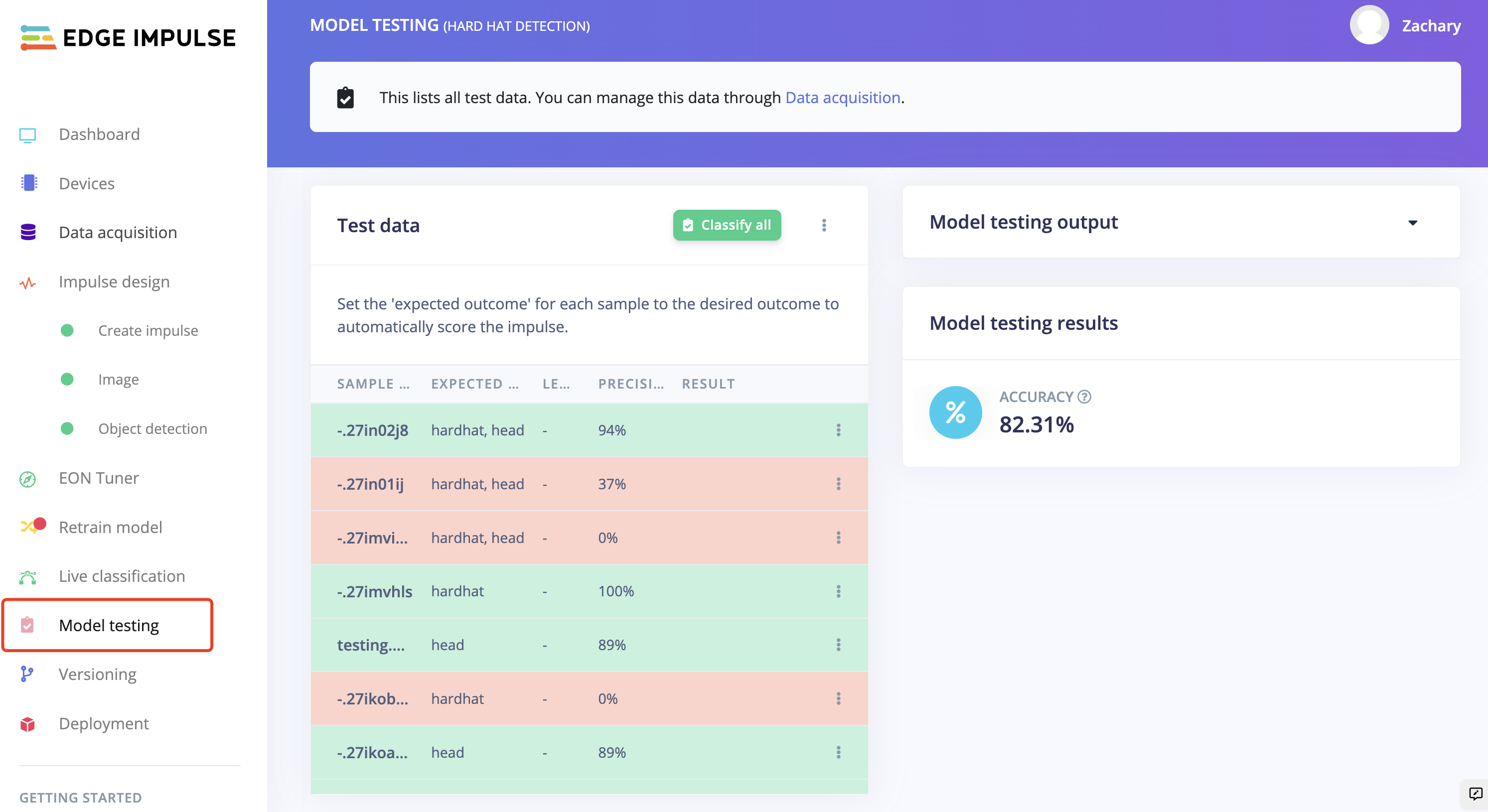
建议混合我们提供的上述方法,并检查每个模型的性能,以查看哪个更好。
将 ML 模型部署到 Jetson Nano
现在我们将把训练好的 ML 模型部署到 Jetson Nano,并应用代码使其运行。
通过 Edge Impulse Linux CLI 部署 ML 模型
- 步骤 1. 根据硬件进行设置 NVIDIA Jetson Nano 开发套件 或 NVIDIA Jetson Nano 2GB 开发套件 用于连接外部显示器和键盘。 将显示屏连接到 Jetson Nano。
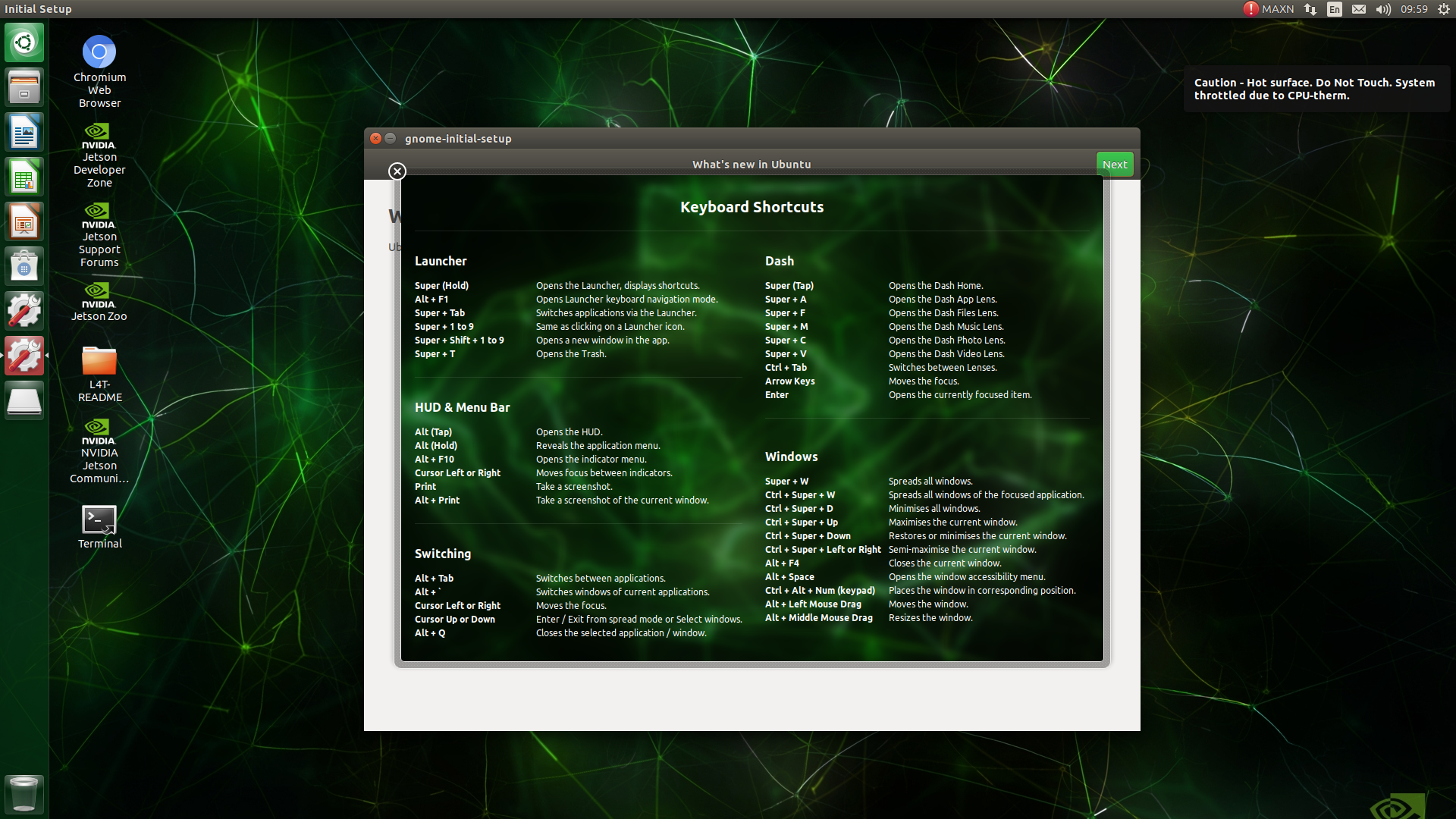
- 步骤 2. 确保您的 Jetson Nano 已连接到互联网,并在 Edge Impulse 中设置设备。
!!!注意 如果您已经在 "基于定制的 NAVDIA Jetson 摄像头数据集的 ML 模型训练" 部分连接了 Jetson Nano,则可以跳过此步骤。
您可以使用以下命令检查网络:
ping -c 3 www.google.com
如果网络正常,结果应如下所示:
3 packets transmitted, 3 received, 0% packet loss, time 2003ms
设置开始运行,使用以下命令:
edge-impulse-linux
网站将请求 Edge Impulse 账户。
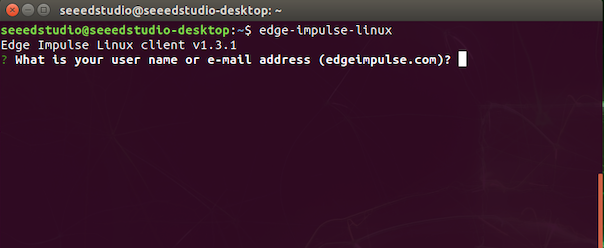
如下所示的内容意味着连接已完成。 我们在 Edge Impulse 中保存的所有项目都是可选择的。
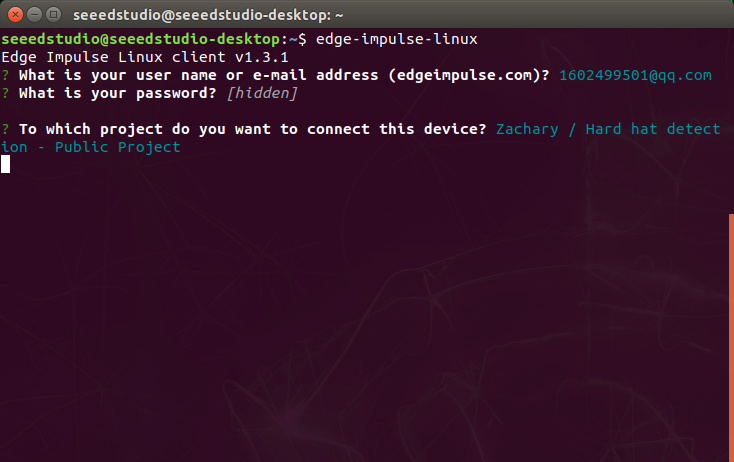
我们正在捕捉照片,因此这里需要选择我们的 USB 摄像头以在网站上应用。
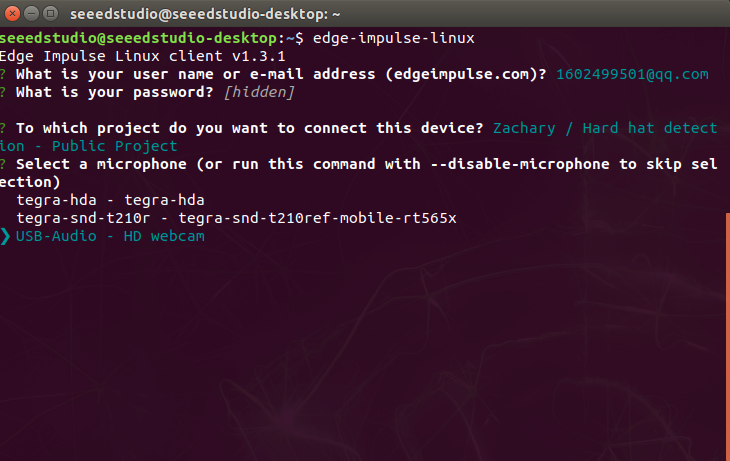
命名我们想要连接到网站的设备
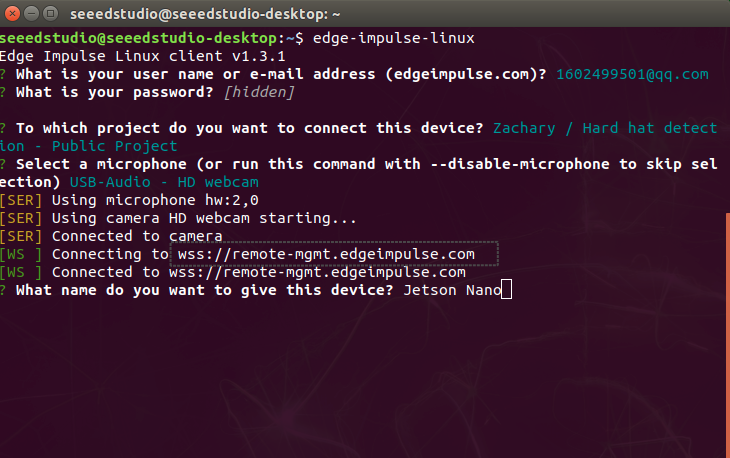
很明显,设备 Jetson Nano 现在已连接到项目。
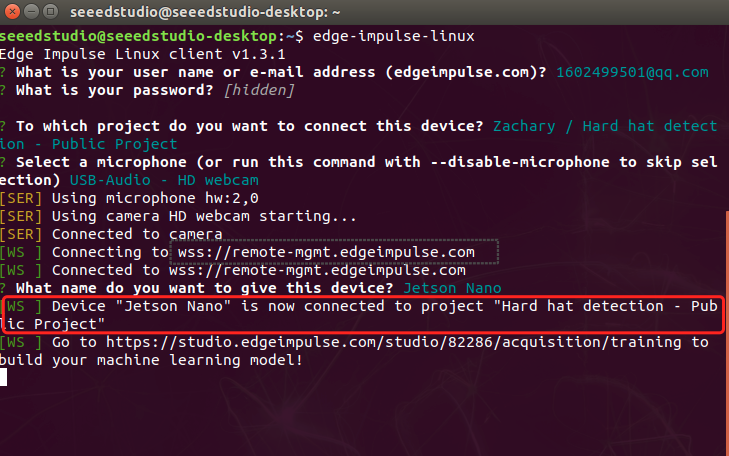
- 步骤 3. 通过以下代码将 ML 模型下载到 Jetson Nano。
edge-impulse-linux-runner
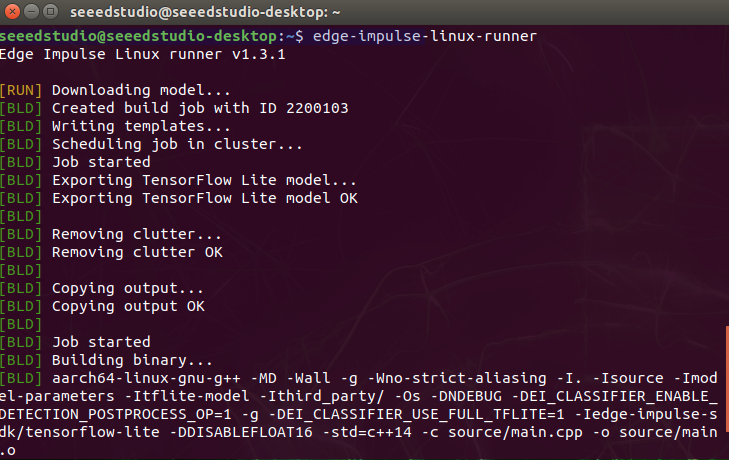
成功连接如下所示,模型将自动激活。
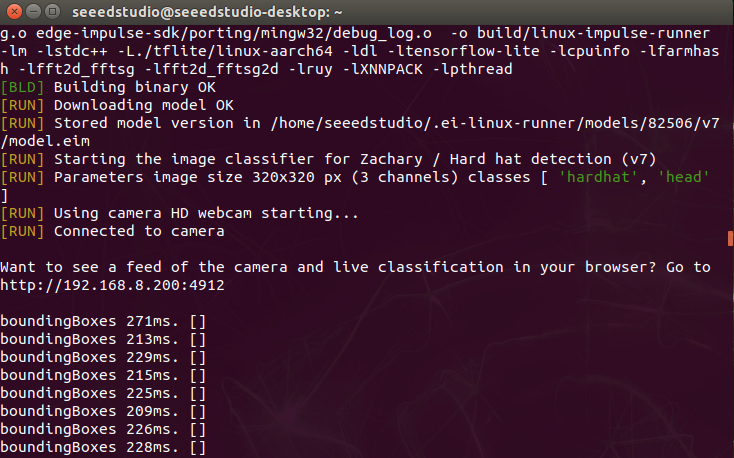
- 步骤 4. 复制显示的地址并在浏览器中打开。
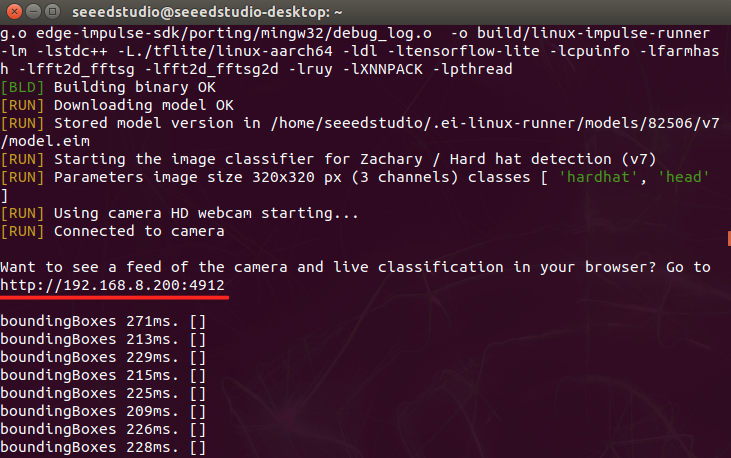
检测结果将在浏览器中显示。
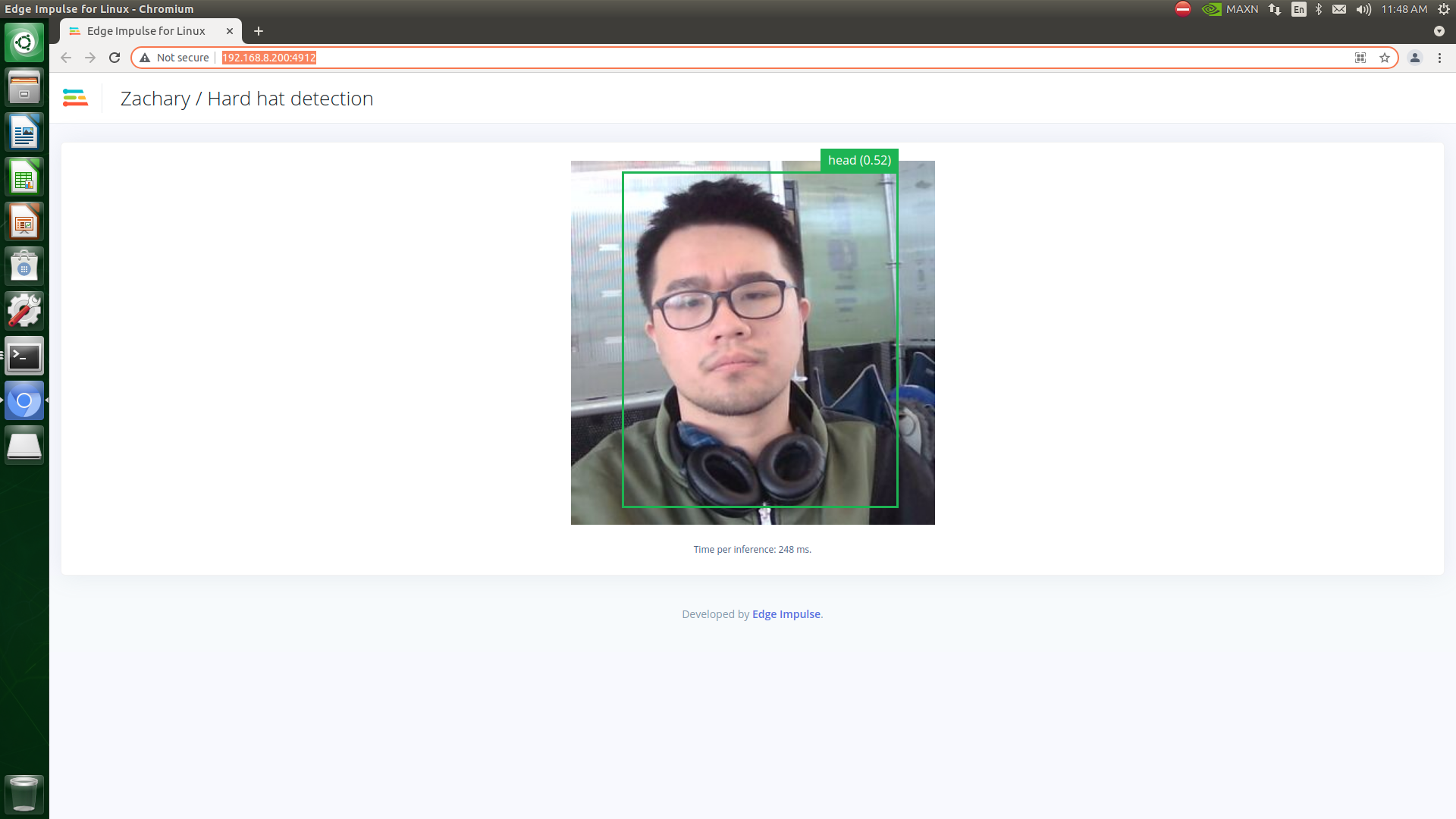
数据结果将如下所示:
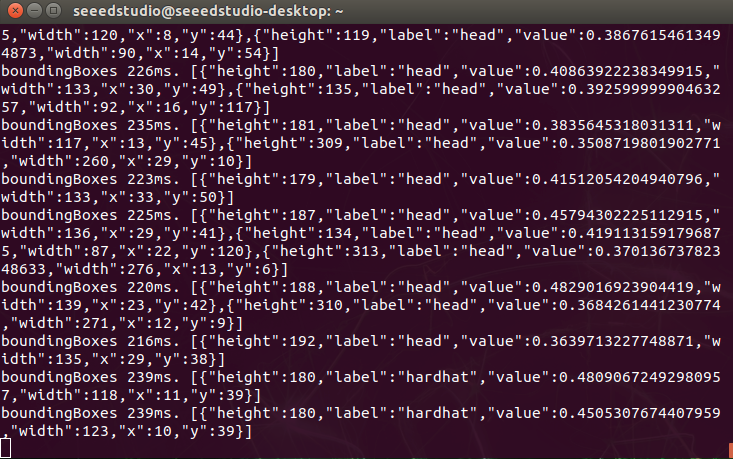
通过 Linux Python SDK 部署 ML 模型
在这个项目中,我们将应用模型在屏幕上显示硬帽的检测,显示 "禁止入内" 和 "欢迎"。 Edge Impulse 提供了一个库,使得在 Linux 机器上使用 Python 运行 ML 模型和收集传感器数据成为可能。该 SDK 是开源的,并托管在 GitHub上。您还可以尝试 镜像 我们已经设置好的。
步骤 1. 安装最新版本的 Python 3(>=3.7) 适用于 Linux。
步骤 2. 使用以下命令安装 Linux Python SDK:
sudo apt-get install libatlas-base-dev libportaudio2 libportaudiocpp0 portaudio19-dev
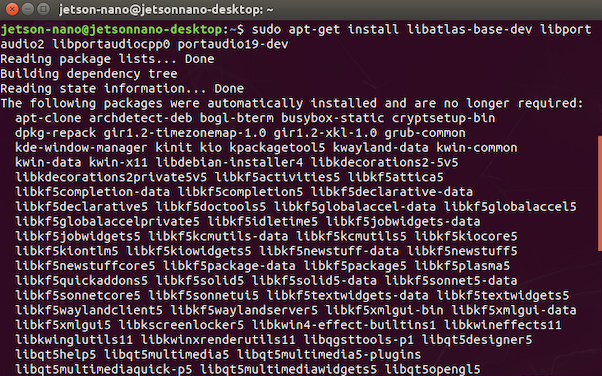
pip3 install edge_impulse_linux
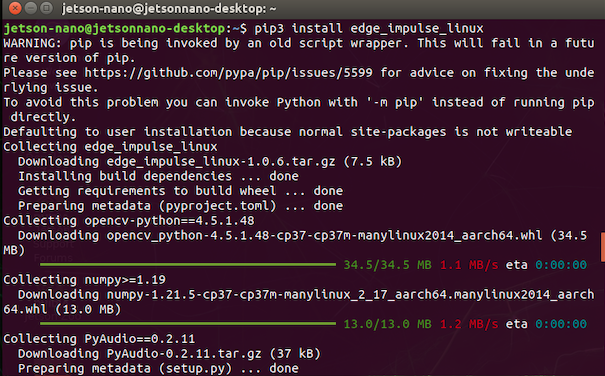
- 步骤 3。安装 Edge Impulse for Linux CLI 使用以下命令:
sudo apt install python3.7-dev
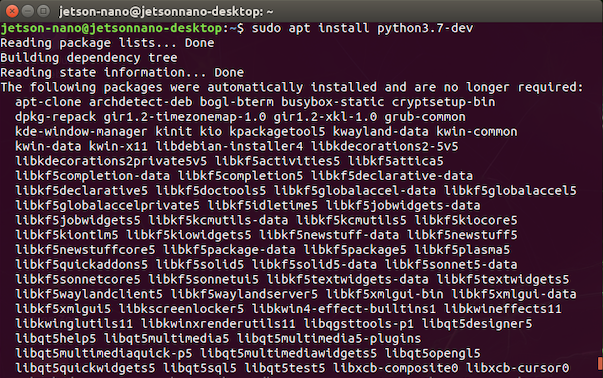
wget -q -0 - https://cdn.edgeimpulse.com/firmware/linux/jetson.sh | bash
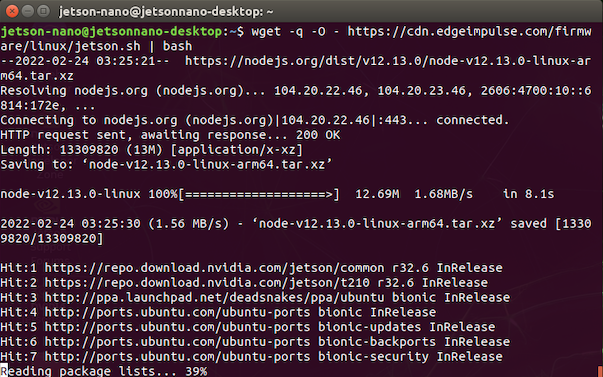
- 步骤 4. 通过以下命令将 ML 模型下载到 Jetson Nano:
edge-impulse-linux-runner --download modelfile.eim
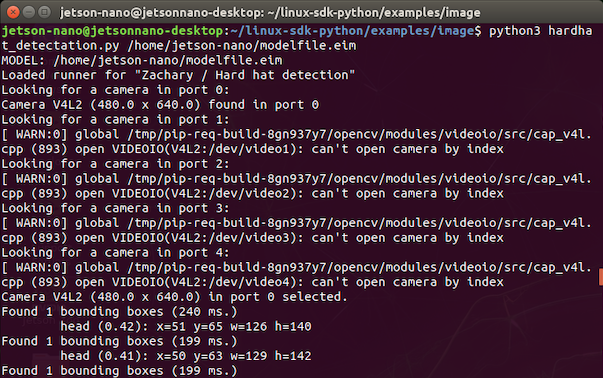
如果这是 Jetson Nano 和 Edge Impulse 之间的第一次连接,网站将要求您输入 Edge Impulse 账户信息以登录。
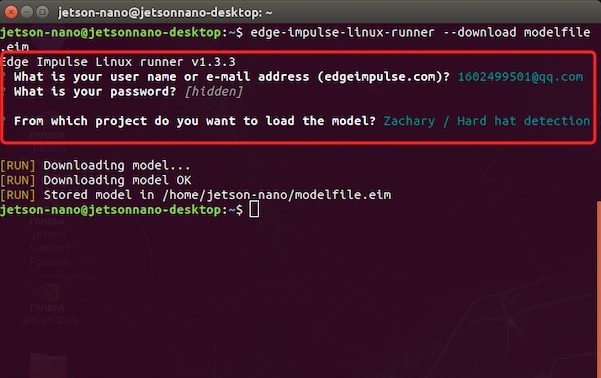
!!!注意 这将文件下载到 modelfile.eim,如果你想切换项目,可以通过添加 '--clean' 来实现。
- 步骤 5运行 hardhat_detectation.py 以使用以下命令应用 ML 模型。 代码可能需要一个外部 文件.
python3 hardhat_detectation.py /home/jetson-nano/modelfile.eim
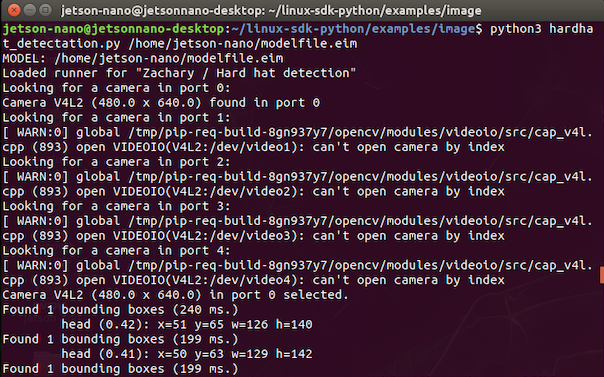
- 步骤 6. 结果应该与这些相似:
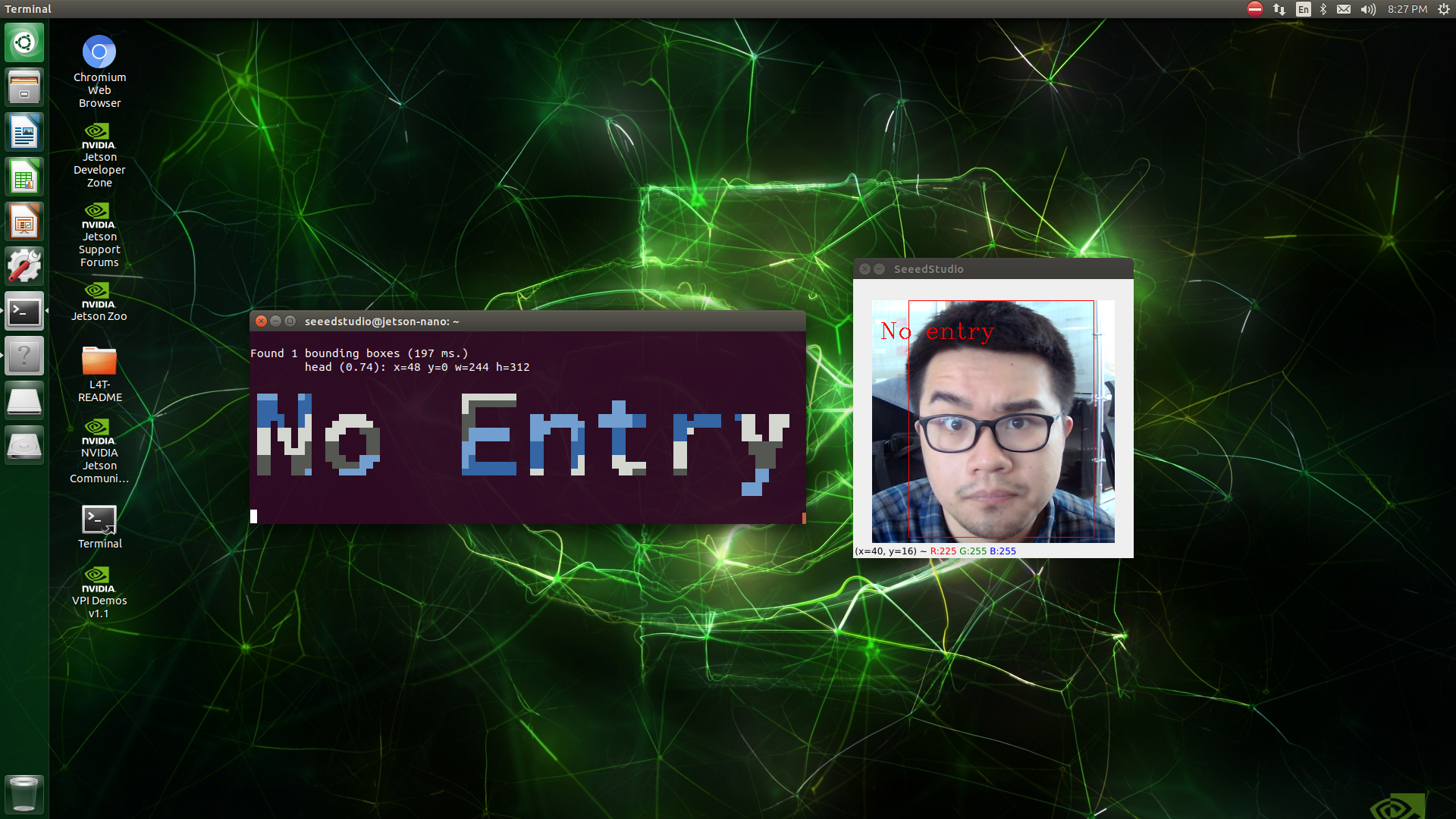
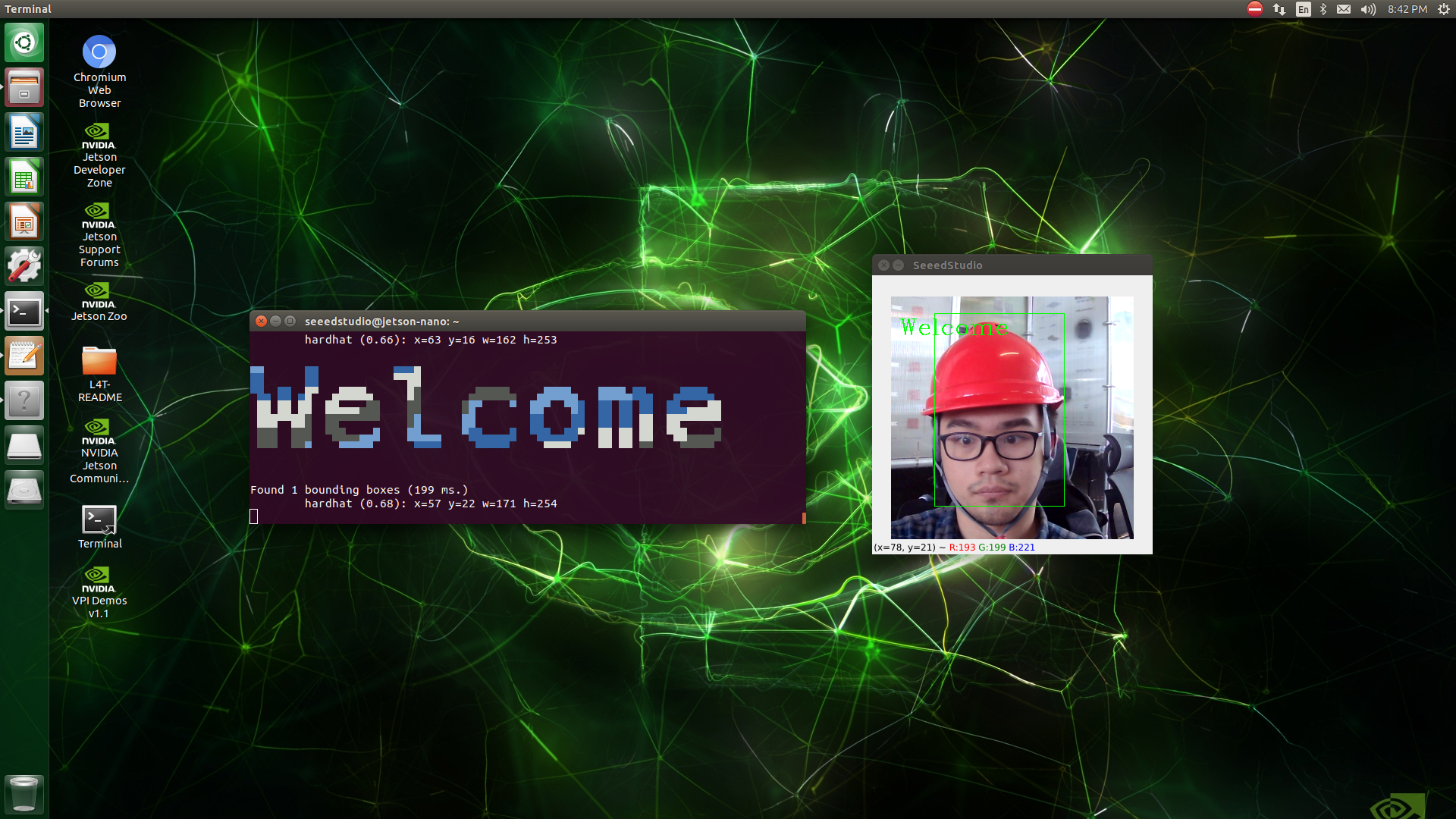
或者它可以是一个图像,您能在 5 分钟内部署您的 ML 应用程序以进行 PPE 检测管道吗?请继续关注我们!
技术支持与产品讨论
感谢您选择我们的产品!我们在这里为您提供不同的支持,以确保您使用我们的产品的体验尽可能顺利。 我们提供多种沟通渠道,以满足不同的偏好和需求。